“One of the main strengths of the current generation of large language models is the ability of their interactive nature to provide a highly competent model that people can interact with and query whatever they want.”
– Philipp Schoenegger
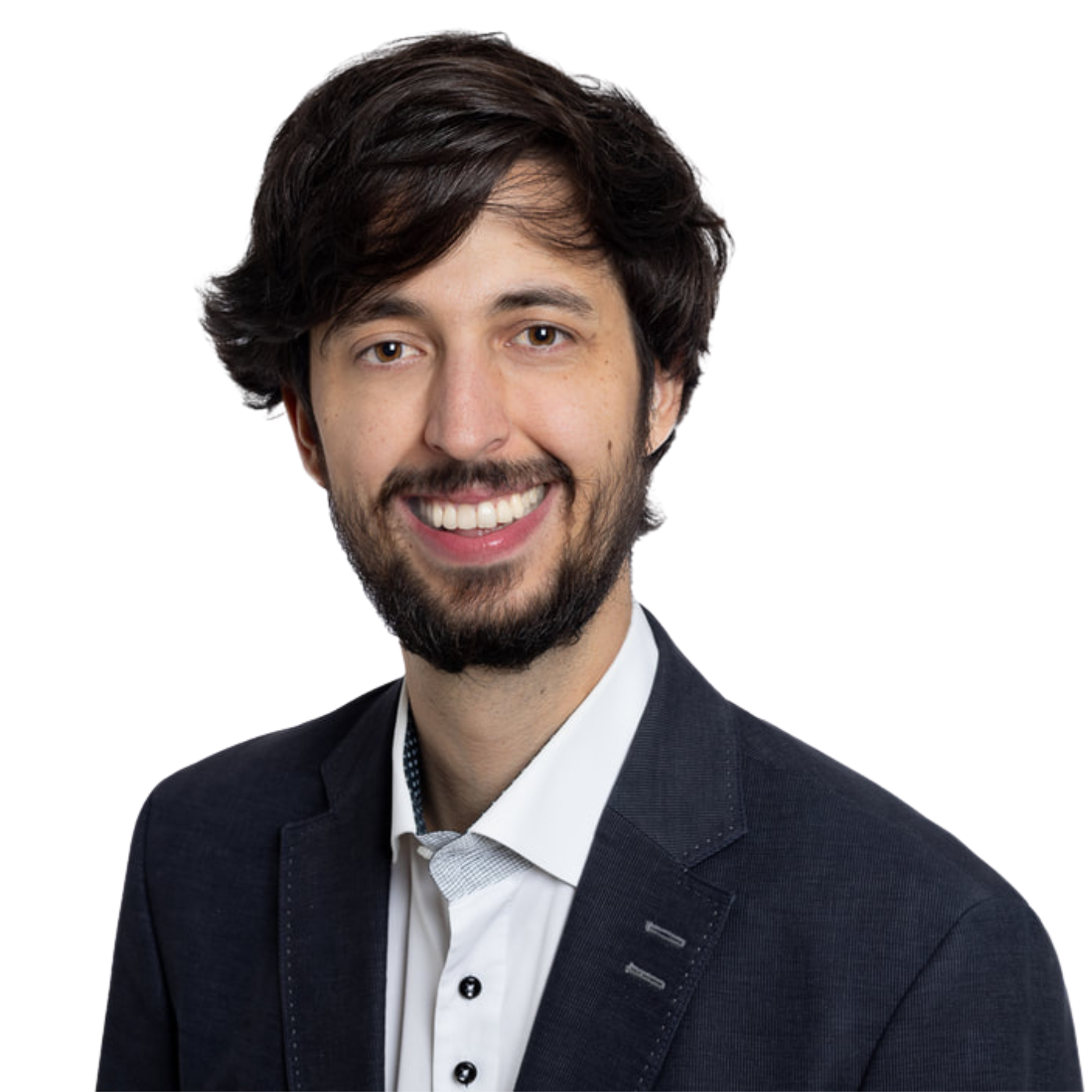
About Philipp Schoenegger
Philipp Schoenegger is a researcher at London School of Economics working at the intersection of judgement, decision-making, and applied artificial intelligence. He is also a professional forecaster, working as a forecasting consultant for the Swift Centre as well as a ‘Pro Forecaster’ for Metaculus, providing probabilistic forecasts and detailed rationales for a variety of major organizations.
What you will learn
- Exploring the intersection of AI and human decision-making
- The catalytic effect of ChatGPT on modern research
- The fundamentals of AI-augmented forecasting
- Unpacking the wisdom of AI crowds
- The journey to becoming a superforecaster
- Navigating the blend of human intuition and AI computation
- Insights into the future of AI-enhanced judgment
Episode Resources
Artificial Intelligence (AI)
Large Language Models (LLMs)
ChatGPT
Judgment and Decision Making
Superforecasting
Philip Tetlock
AI Augmentation
The 10 Commandments of Forecasting
Alibaba
Claude (Language Model)
Palm (Language Model)
External vs. Internal View in Forecasting
International Energy Agency (IEA)
Metaculus Platform (Forecasting Platform)
Papers
AI-Augmented Predictions: LLM Assistants Improve Human Forecasting Accuracy
Wisdom of the Silicon Crowd: LLM Ensemble Prediction Capabilities Rival Human Crowd Accuracy
Transcript
Ross Dawson: Philip, it’s wonderful to have you on the show.
Philipp Schoenegger: Thank you so much. Thanks for the invitation. It’s great to be here.
Ross: So on your website, you have this very interesting diagram, which shows that your current research is around the intersection of judgment and decision making, and applied artificial intelligence. So what is that space? And how have you come there? What’s, what is that that pulled you to this particular space?
Philipp: So I think what, what really motivated me to work in this area is what motivated many other people to jump into AI. And this was just the release of ChatGPT, in late 2022. So I hadn’t been working in artificial intelligence before, I have a social science and humanities background, having worked on charitable giving, and political philosophy before. But having seen ChatGPT, I think it took 10 days. Until I had my first research project, we’ve caught up slowly. And our first idea was, how can we? How can we kind of mimic social science participants with artificial intelligence? And so what we did is we ran a bunch of studies that had been replicated in humans with the text DaVinci, free to see the early ChatGPT model. And ever since I’ve never looked back, and I’ve pretty much only wanted to do more AI stuff. It’s way too interesting. At this point, pretty much almost all the work.
Ross: I think we’re pretty aligned on that. And it’s just like this intersection of human intelligence, artificial intelligence is so deep, so promising, so much potential. And so now it’s wonderful to see the work that you’re doing. So speaking of which, we recently, you were a lead author of a paper.
AI Augmented Predictions, LLM assistants improve human forecasting accuracy. So first of all, let’s just describe the paper at a high level, and then we can dig into some of the specifics.
Philipp: So the basic idea of this paper is, how can we improve human forecasting. Human judgmental forecasting is basically the idea that you can query a bunch of various interests and sometimes lay people off about future events, and then aggregate their predictions and arrive at surprisingly accurate estimations of future outcomes. So this goes back to the work on Super forecasting, the Philip Tetlock.
There’s a lot of different approaches on how one might go about improving human prediction capabilities, absolute maximum training, as it was called the 10 commandments of forecasting, how you can better forecast out or there might be some, some, some conversations where different forecasters talk to each other and exchange their views. And we wanted to look at how we could think about improving human forecasting with AI? And I think one of the main strengths of the current generation of large language models is the ability of the interactive nature of the back and forth to have a highly competent model that people can interact with and query whatever they want. Really, they might ask the model, ‘Please help me with this question. What’s the answer?’ They might also just say, ‘Here’s what I think please critique it.’ And so this opens up for human focus, like a whole host of different interactions. And we wanted to see what the effect of this might be on forecasting accuracy.
Ross: That’s fascinating, I suppose one of the starting points is thinking about these forecasters. So I suppose just so people could be clear that human forecasting in complex domains is superior to AI forecasting, because they don’t have those capabilities. So they’re saying humans are better than AI alone. But now the results of the paper suggest that the humans augmented by AI are superior to either humans alone or AI alone.
Philipp: At the current amounts of papers that I have published, yes. But depending on when this airs, there might be another paper coming out that adds another twist to this. But yeah, so in early work, we find that that just a simple GPT 4 forecaster underperforms, the human crowd and on top of added underperforms, just seeing 50% on every question, but in this paper, we found that if we give people the opportunity to interact within large language model, which in this case was TBD for turbo, and we prompted it specifically to provide super forecasting advice.
So our main treatment had a prompt that explained that the 10 commandments are super forecasting and instructed a model to provide estimates that take care of the base rate, so you look at how often is it that things like this have typically happened, that quantifies uncertainty that identifies branch points in reasoning. But then we also looked at what happens if the large language model doesn’t give good advice? What if it gives what is called bias, that is, if I’d be more noisy advice. So what if the model is told to not think about the base rate, so not think about how often things happen to be overconfident to basically give very high or very low estimates to be very confident. And, to our surprise, we find that actually, these two approaches similarly effectively improve forecasting accuracy, which is not what we expected.
Ross: I think that this is a really interesting point, because essentially, this is about human cognition. So it is human cognition, taking very complex domains, and coming up with a forecast of a probability of an event. So or a specific outcome in a defined timeframe. So in this case, the interaction with the AI is a way of enhancing human cognition, that they are basically making a better sense of the world. And I guess one of the things which is more distinctive about your approach is, as you say, you could allow them to use anything anyways of interacting, as opposed to a specific dynamic. So in this case, it was all human directed. There was no AI direction. It is AI as a tool, with humans, I suppose, seeking to augment their own ways of thinking about this challenge.
Philipp: That’s right. And of course, being human has asked me to, like make at least a sizable amount of participants just simply ask them the question, right. I just said, well, once the question will be the closing value for the Dow Jones at the end of December, and I just copied it in and just saw what the model did. But then many others did not. And they had their own view. And they typed in, ‘Well, I think that’s the answer. What do you think?’ Or, you know, ‘Please critique this.’ And I think these kinds of interactions are especially promising going forward, because there’s also this whole literature on the different impact of AI augmentation on differently skilled participants, differently skilled workers.
In my understanding, the literature is currently mixed. So studies are finding different results. So we didn’t find a specific effect here. But other work finds that when the model just gives the answer, low performance typically tends to do better, because you know, they’ll know the answer. And the models are probably better than them. But if the model is instructed to give guidance, only low performers tend to, you know, not be able to pick up on the guidance and follow it. But I think there’s still, there’s still a lot of interesting work to be done before we can pin this down, because there’s so much diversity in which models are being used. What’s the context?
Ross: Yeah. But I think that’s a particularly interesting outcome in the sense that humans are mainly not very good forecasters. And it’s only a relatively small proportion of people who are good forecasters. So it would have thought that there would be some kind of differential because it’s almost like, people who have some kind of understanding of what forecasting takes, and others who doubt the kind of basically, you’re guessing, but it showed some similar improvement. I think that’s a very interesting outcome.
Philipp: Yes. So of course, it might be that the reason we see similar improvements is that we group the same population into high and low skilled groups based on a different test, and that the effect might be vastly different if you pick a random subset of the people. And then people who do forecasting for a living like truly high skilled forecasters.
I think it’s very plausible that the effect here is different. But most studies just take the same batch of employees or workers or study participants, and then divide them by some type of criterion, which is what we also did. And yeah, we did not find an effect. And similarly, we didn’t find the disparate effect on question difficulty as well. So we expected that, maybe participants are more likely to just defer to the AI on hard questions and easy questions to do it themselves or something different. And there was also no significant effect as well.
Ross: Right. So you mentioned before that the subjects would use the AI very differently. So it may not have been specifically part of your research. But do you have any indications of the types of ways in which using the AI created the most value or augment the decision making or forecasting the most?
Philipp: I wish I did. I looked into it. I just struggled to come up with a very strong and defensible method, especially after having seen the data. So I typically like to write down exactly what I’m going to do before I see the data to kind of avoid a contamination of what I think I should do with the results. But I think at least on some questions, people just didn’t seem to benefit from getting an anchor. Some of the questions are really difficult. There were like, you know about Bitcoin hash rates, commercial flights on a certain day. That’s not something one has a type of intuition about. I don’t know how many flights are there, globally, or at any given day, especially at the end of December, I could be off by orders of magnitude.
And I think one of the most simple, helpful ways to model can help is just give a prediction that is within one order of magnitude most of the time. So it’s a starting point. Yes, that’s right. I’m talking 10s of thousands, or millions, or like, what are we talking about? And I think, especially on like, harder questions, those were, I think, harder questions generally, like, ‘How many AI papers will be published in a given month,’ It’s difficult, difficult to know if I was researching. And I think one big improvement here is simply the speed where, of course, people could go online, they could search the terms, they could try to find a source, they could double check it, but it will take half an hour to an hour. What was the simple interaction of the model in seven seconds?
Ross: And is one of the other ways as you said, interrogating the AI? So in the sense ofI suppose a couple of frames. One is, you know, ‘This is what I’m thinking, Do you have any other ideas?’ And the other one is around identifying different criteria, which may affect the outcome which the person may not have considered?
Philipp: Yes, absolutely. We didn’t see this in the majority of interactions, but there are definitely people who did use it like this. And I think especially once you move to more sophisticated contexts, where people have, like a higher investment in the outcomes, I think this will most likely be the other kind of margin, at least, the most successful way to be augmented by to have the back and forth of one’s own kind of use and points but and also take the outside opportunity to see like a model prediction and get feedback on one’s own arguments.
Ross: So you’ve already spent a moment describing the super forecasting prompt that you use for the research. And so it’s quite a long prompt, as you say, it mentions the 10 commandments of super forecasting, and provides quite a lot of detailed guidance on how to interact and describe probabilities and so on. So I’d be interested to know how you came up with that particular prompt? Did you try many types of prompts? What was the kind of testing to be able to provide this as an optimal super forecasting prompt?
Philipp: Yes, so it’s clearly not optimal, so that they didn’t run independent and critical analysis to make sure this is indeed, the most optimal. But I think the first step that anybody who tries to interact with is about forecasting, especially a couple of months ago, really was that many models just did not want to give forecasts for the future. And they had, like an aversion it’s unclear at which point of the model pipeline this was introduced, but an aversion to providing probabilities about future events, they were generally very hesitant to give probabilities, or even specific quantities as a study.
So the first part was simply drawn from a previous paper where we spent a lot of time trying to figure out how to consistently get GPT to give a forecast. So this has to work, you know, simply asking, just like naively, that doesn’t work. And then we basically drew on the literature of super forecasting and tried to supplement that approach with what we thought in humans would be the most appropriate and most promising approach to think about future events.
Ross: So have you tested a variety of different types of prompts?
Philipp: We’ve tested a variety of different types of prompts on the outcome, complexity and helpfulness, not accuracy. The main idea here wasn’t to get the most accurate forecast. The main idea was to get if you’re, if you’re prompted, respond in the way that we would like a super forecasting assistant to respond, right? If you’re in for a prediction? Do they give you a prediction, and also give you the reasons for and against if you ask them now to explain. Do they give an explanation if you give your forecast to take the ticket? So yeah, we will get like a trial of a bunch of different prompts to see which one most mimics the type of assistant behavior we thought would be most useful for our treatment.
Ross: I am just interested, have you tested the other – the major, large language models to see if there’s any differences in their propensity or ability at forecasting?
Philipp: This might be I don’t know when this episode will air so the paper might be out by then. But there’s a paper where we do exactly that. So what I call the Wisdom of the Silicon Crowd paper, which is where we try to mimic human cloud forecasting, via 12 distinct language models that are very diverse that are interconnected Quinn, seven B, from Alibaba, and of course GPT4 and Claude, and Palm and everything else. And we have every model give several forecasts on over 30 questions, and then we aggregate them. And then we actually find that the crowd of MLMs matches human forecasting performance. And this is the first time I think, this is salt has been found that if the large language models themselves form a cloud, they can they can hit the gold standard of a human forecasting tournament, which is even higher, because it’s very interesting experience people forecasting there,
Ross: Extremely interesting. So in that case, was the aggregation a simple mean, or what is the structure for aggregating the different models predictions?
Philipp: This is one of my favorite findings in the forecasting literature generally, is that like, yes, there’s many ways to do really fancy aggregation methods. But a simple median is extremely powerful. And this is literally So the median is not the average. It’s just taking the mean, right? Yep, just a median. And this is just extremely powerful across different contexts across different deviations from ideal scenarios.
And that’s also what we use here. And, of course, there’s massive heterogeneity. So diversity and how well models do some models do really badly. Don’t call it the worst one. But so some models are very prone to forecasts of 99% or 1%. Right? They just think, like things happen or don’t happen, whereas other models are more in the middle. And we also find that across the large language models, what is possibly something like what’s called an acquiescence effect, which is the effect that whatever the question is, the model is more likely to say Yes, than No. It doesn’t matter what the question is. And we find that the cloud overall is more likely to be on the side above 50% on the forecasts, despite the fact that less than 50% of questions that solve positively. So there is really a bias in that response. But nonetheless, the cloud effect still matches human accuracy and exceeds a simple benchmark of just giving 50%.
Ross: Extremely interesting. So, you mentioned this in some of your papers, but I mean, just what we’ve already touched on them in a way, but what are sort of short term and medium term research directions in the space of forecasting and decision-making and augmented with AI?
Philipp: So that’s, that’s a lot of things happening right now. Many, many people are working on this. I think what I’m most interested in working on is currently the AI plus human and human plus AI interactions. And to see like, these first papers were like a stab at it to see. Yeah, the effect is real, it works. But now I think there’s a lot of work to be done to more closely and more specifically look into what exactly is it that improves these performances. So for example, we like the paper we discussed first, it was humans being augmented by AI. In the second study on a different paper, AI is being augmented by humans in a way. So we have AI that predicts a bunch of different effects. And then they are being told what the human set says about these topics. And then they’re being told, ‘Well, here’s new information for you. A human tournament gives this 45% chance, you are now free to update however you want.’ And we find that actually the AI predictions get significantly more accurate, after learning.
Ross: Fantastic afternoon.
Philipp: But there’s a small caveat. This effect of improving the accuracy is less effective than if one had just taken the machine forecast and the human forecast and averaged them. Right. So there’s still a bias in the model towards, towards I think its own views, and it only updates somewhat towards the human. And it doesn’t properly distinguish between when the humans might be better than them and when they might be better to be, you know, relying on their own predictions. So you know, there are improvements, but they’re still not above simply averaging. And I think just getting that right getting the what read improves model performance from humans, what improves human performance from AI? Is it numbers or is it maybe maybe it’s not numbers at all? So I’m especially interested in seeing just very fancy and complex rationale. So reasoning for forecasts without having the numbers to see if that can improve performance, because that would eliminate the worry that people are just copying the new model.
Ross: Yes, yes. There’s a lot of rich aspects to that, including what are the mechanisms and structures for bringing together human and AI insight and sequencing and structure. But as you say, it’s often the simplest that can come up with the best result.
So switching gears a bit you are, amongst other things, a professional forecaster. So you’re one of these humans, you’re at the high end of the spectrum, in having skills, techniques, capabilities and performance, which exceeds others in being able to forecast extremely complex events. So what do you think about this? How have you developed this capability?
Philipp: That’s a good question. I think the first caveat here really has to be that having worked with so many other experienced professional forecasters, there is no one size fits all answer, I think, we share some characteristics and backgrounds and methods. But I think in many ways, we are quite distinct from each other. So historically, the way I started it, I just got extremely interested in forecasting after the COVID pandemic, because I was so good at forecasting the very beginning and so bad at forecasting the middle, that I had two data points of like a great success and a great failure. And I was like, well, am I good at this, I’m really bad at this. And so then I signed up to one of those platforms, metaculus to get basically a track record.
And so I went on, hundreds and hundreds of thousands of predictions at this point. And I think the main thing that really helps, is the distinction between outside and inside view. So if people haven’t heard that before, the inside view is basically one’s personal opinion about things. So if I just think about the chance of Donald Trump winning the presidential election in November, I might have my own kind of use. But then there’s the outside view, which is a map view polls, better people that might be accurate forecasts. That might be track records that might be how likely a person is to be a president who didn’t win the election, likely to win the election after that. And I think the most important thing in forecasting is not to not stick too hard to both ones inside view. And also not to always defer to the outside view. So I think the main challenge here is to find this balance between where, yes, actually, I probably should just defer to what other people are saying. And also to be on this kind of point of view, I think you have an advantage that really adds to it. And I should stick to my guns and be like 10% above or below? What would be the you know, the baseline expectation.
Ross: I’ve taken, for example, Donald Trump being elected president at the end of this year, so how would you go about it? I mean, is there a sequence of things where you consider the different factors or you take an external input? So or do you build a sort of a structured process to be able to start and then get to a point where you have a forecast?
Philipp: For exactly this question, a second in response to the previous question. First, one feature of experience forecasts is also knowing when not to forecast, and I was invited to a project on a Donald Trump election. And I chose not to forecast on this, because I thought I didn’t have a good enough procedure to add, like a lot of expertise and forecasting accuracy. And so actually, for questions where one probably doesn’t have a good edge, but doesn’t have like an additional part of knowledge, or a good track record, or a really high level view of all the information, it’s probably best to not forecast, like it’s an easy way to jumping into things that I’m probably isn’t best suited for.
Ross: So let’s say it is a subject. So whatever subject is something which is in your area of expertise, or you feel you have something to add. So at that point, do you have a process or approach in working through this challenge?
Philipp: Perfect, yes. Step one, for me, is to always get a very broad view of what everyone else is thinking. So part of the reason I enjoy forecasting so much is because one gets to work on so many different topics from Chinese coal consumption to climate change to financial markets. So the first thing I do is to try to read as much as possible, and to get as many forecasts predictions and rough numbers of base rates in this context as it’s possible so often, when the thing one might want to forecast isn’t quite the same that what other people are working on, but just to get a rough picture, and then to basically kind of construct what would the number be like if it just continued as usual? So what’s the actual trendline? And then to ensure that deviations from this trendline have to be justified to myself, quite specifically?
Because, very often the future is definitely like the past, of course, sometimes it absolutely, it’s not. And those change points are very hard to forecast. And also, most experienced forecasters build a track record in environments where yes, the future is, is something like the past, we can sample, we can’t sample the AI revolution a thousand times and see who gets it right. We can only sample repeated elections and economic indicators. So I think my bias here really is, you know, trend continuation as a first step. And then try to identify biases in individual people who might hold that trend, or who might argue for deviations of that.
Another kind of thing to look at is when evaluating sources, try to go back to those sources, previous views and predictions, so often, they’re in there, but something like the International Energy Agency. Sometimes they do publish the forecasts, and I forgot what the type of graph is called. But it’s a very, very striking graph for interest rate predictions, where the actual interest rate and the predictions at every year five years out, are shown and and I think it’s called, like a hair haircuts diagram, something like that, where the predictions get it wrong, almost every time and to basically try to identify where those biases exist, and in what direction they are. They’re optimistic about climate change, are extremely pessimistic, and then try to kind of account for the underlying bias in the trend. And that I think gives me a first kind of basis. And this can be done via…you can do your own time series, modeling some machine and stuff. But it can also be purely judgemental, just like in terms of numbers, especially where there isn’t much data to go on – one can fit a model to something where it has like, three data points.
Ross: Yeah, a couple, I mean, a couple of points there. One is that if you are starting from other people’s forecasts, I mean, basically, there aren’t very many good forecasts out there. They’re either commercially biased, or they’re just not what many people are really trying to do and publish as forecasts. So there’s not actually a lot out there. But it’s another jittering point saying, you start with other people’s forecasts, rather than sort of starting from the inside view, Full Movie, the outside view. So I suppose at that point, what you’re trying to do, as you say, is to find what are all the failures of the existing predictions, you can add some value. That’s right.
Philipp: That’s right. And that’s, of course, other people I’ve worked with, will do quite the opposite, they have to have their own view about how the world works. And then that standard inside view, and then use the outside view to supplement it. But I think this is, this is not the way I operate. And of course, they’ve been successful in doing so. But I really think that one can learn a lot from actually reading all the data and getting all the insights from all the different areas, especially on most projects. I work on it for 20,40, 50, 100 hours only, for a whole kind of context. And I think one will miss a lot by just going on intuition, because, you know, my intuitions are in my current contexts, decent probably. But there’s a lot of things I don’t know about. And I think it’s very good to continue to be humble, and to just try to get a trendline going and stick to something like that.
Ross: So let’s say you’re speaking to somebody who just needs, you know, be useful to make better predictions in their work. Leader – business leader, startup leader, whatever. So what will be our advice, what are just a few things that they should start doing, which will make their predictions that better than they used to be.
Philipp: One advice is just don’t think you’re too special and get a view of the base rate of a trendline. And the second thing is, try to find a way to get experience forecasts with good track records to help you. So this can be by a business that offers like, like a swift center I work for, but this can also be internal. So this could just be an internal forecasting competition on the stuff that really matters to your business. Keep a track record of this and try to identify over months and years who’s like the three best people we have on this and then make sure to draw on them going forward.
Of course, this is very risky, because these types of internal competitions can end seniority hierarchies very quickly, if a junior analyst who stayed out of undergrad just turns out to be better than everyone else. But I think just identifying the people who actually could do this consistently. And in the context you cared about, I think it could be very useful for most businesses that have at least medium size, what I can kind of think about holding an internal competition like this.
Ross: Yeah, I think that’s a really good idea. I did recalls, one of the early enterprise crowdsourcing examples was Google used for sales forecasting. So essentially, using a crowd, they found that, significantly better than all of the sales forecasts they had.
Philipp: So there’s a lot of interesting work right now on our prediction markets, better forecasting tournaments. And I think, you know, many people might get sidetracked with what should focus too much on this, but as the recent work just shows that actually, the most important thing is just getting the experienced forecasters, it doesn’t really matter. If it’s a prediction market or forecasting platform with just just a monthly survey. I think the biggest bang for the buck really is identifying the people who were most equipped to forecast that event rather than the context of any business organization.
Ross: Fantastic. I will certainly be following your work closely. I think it’s fascinating; a really interesting paper. Sounds like the one which is about to come out. I’ll definitely be diving into that. So love your work. Thank you. Is there anywhere people can go to find out more about what you’re doing?
Philipp: Thank you so much. So I’m mostly on Twitter, on social media and also my website. But I think Twitter and websites are probably the best. The best spot.
Ross: Right? Fabulous. All those will be in the show notes. Thanks so much.
Philipp: Thank you so much.
Podcast: Play in new window | Download