“We have unique capabilities, but it’s crucial to understand that today’s AI technologies, powered by deep learning, are fundamentally different. We need a new paradigm to figure out how we can work together.”
– Mohammad Hossein Jarrahi
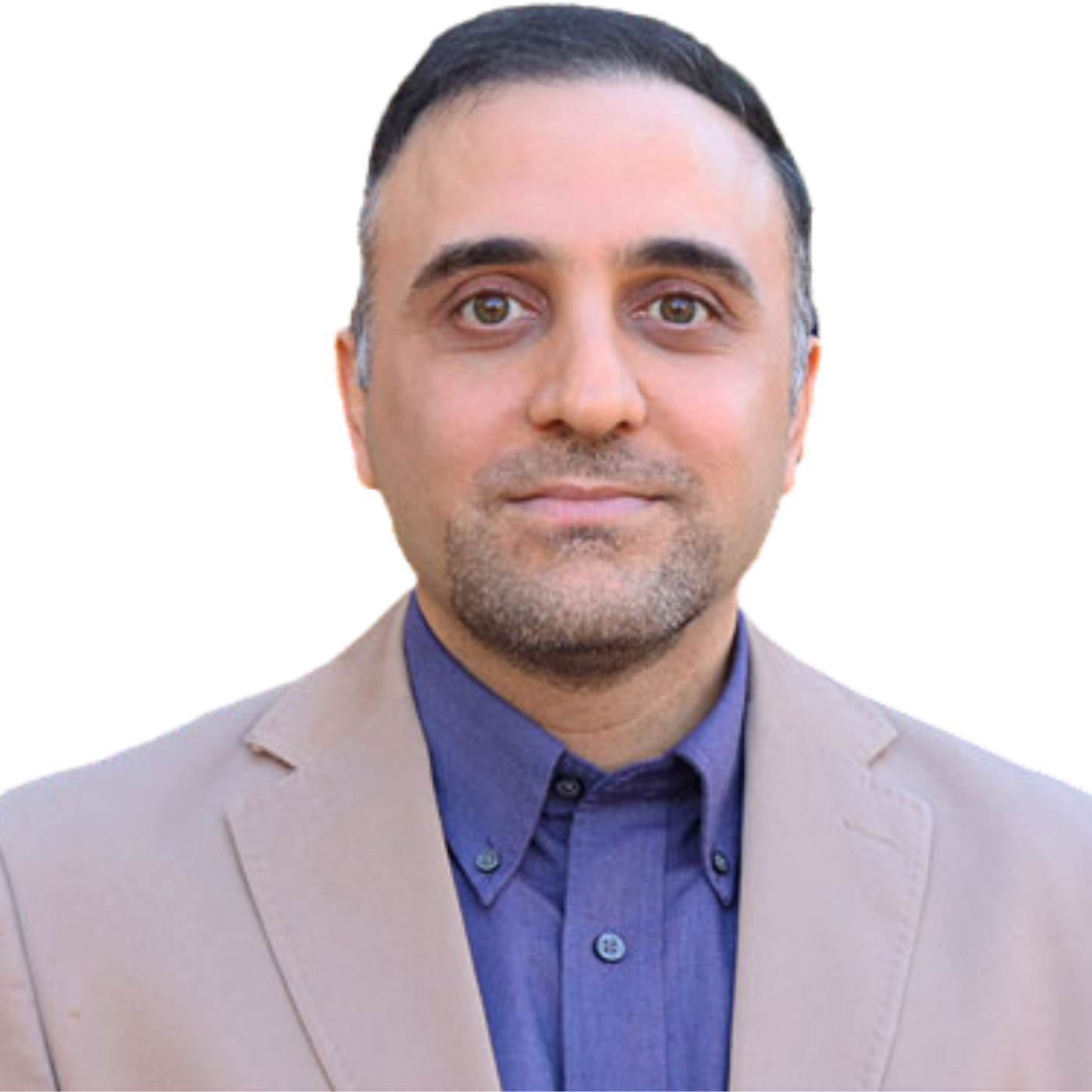
About Mohammad Hossein Jarrahi
Mohammad Hossein Jarrahi is Associate Professor at the School of Information and Library Science at University of North Carolina at Chapel Hill. He has won numerous awards for teaching and his papers, including for his article “Artificial intelligence and the future of work: Human-AI symbiosis in organizational decision making.” His wide-ranging research spans many aspects of the social and organizational implications of information and communication technologies.
Website: Mohammad Hossein Jarrahi
Google Scholar Profile: Mohammad Hossein Jarrahi
LinkedIn: Mohammad Hossein Jarrahi
Article: Artificial intelligence and the future of work: Human-AI symbiosis in organizational decision making
What you will learn
- Exploring the concept of human-AI symbiosis
- Understanding AI’s role in automation and augmentation
- The difference between intuition and data-driven decision making
- Why AI excels at repetitive, data-centric tasks
- The importance of emotional intelligence in human-AI collaboration
- Balancing efficiency and innovation in AI applications
- Building mutual learning between AI systems and humans
Episode Resources
- IBM
- NPR
- ChatGPT
- deep learning
- Skype
- Human-AI symbiosis
- Harvard Business Review
- Turing test
- algorithmic management
- machine learning
- data provenance
- Mayo Clinic
- natural language processing (NLP)
- “Man-Computer Symbiosis”
- intelligence augmentation
People
Articles
Artificial intelligence and the future of work: Human-AI symbiosis in organizational decision making by Mohammad Hossein Jarrahi
What Will Working with AI Really Require? by Mohammad Hossein Jarrahi, Kelly Monahan and Paul Leonardi
Transcript
Ross Dawson: Mohammed, it’s wonderful to have you on the show.
Mohammad Hossein Jarrahi: Very glad to be here.
Ross: So you have been focusing on human AI symbiosis. I’d love to hear how you came to believe this is the thing you should be focusing your energy and attention on,
Mohammad: I was stuck in traffic, 2017 if I want to tell you the story. And this was a conversation between an IBM engineer, and it was on NPR, and they were asking him a bunch of questions about, what is the future of AI like? And this is still before a lot of chatgpt, and the I would call it consumerization of AI, and it clicked. When you’re stuck in traffic, you don’t have much to do. So that was really the moment that I figured out he was basically providing examples that fit these three categories of uncertainty, complexity and eco locality. I went home immediately and started sketching the article and wrote the article in two weeks. But the idea was, we have very unique capabilities. It’s a mistake to underestimate what we can do, but also understanding that these technologies, the smart technologies that we are witnessing today, at that time, were very empowered by deep learning. They’re inherently different from the previous information technologies we’ve been using. So it requires a very different type of paradigm to understand how we can work together. These technologies are not going to make us extinct, but they shouldn’t be thought of as infrastructure technology like Skype, you name it, communication, information technologies have been used in the past in organizations, outside of the organization. So I figured this, this human AI symbiosis terminology, which comes from biology. It’s a very nice way to understand how we as two sources of intelligence can work together.
Ross: Yeah, also very aligned, of course, with my work and people I engage with. I suppose the question is, how do we do it? There’s too few, but quite a few who are engaged in this path. So what are the pathways? We don’t have the answers yet, but what are some of the pathways to be able to move towards human AI symbiosis?
Mohammad: I think we talked about this a bit earlier. It really depends on the context. Now, from this point on, that’s really the crux of issues in my articles I’ve been writing. It really depends on a specific organizational context, how much you can delegate, because we’ve got this dichotomy, which is not really dichotomy. They’re all intertwined, automation and augmentation. Artificial intelligence systems provide these dual affordances. They can automate some of our work and they can augment some of our work. And there is a difference between the two concepts, automation is like doing it somehow autonomously, with a little bit of supervision. Augmentation, we are very involved. We are implicated in the process, but they are just making us more efficient and more effective. You can think about many examples. It really depends on how much automation and augmentation goes into a specific context. For example, in low stake decision making, you’ll see more of automation. A lot of mundane tasks can be offloaded to algorithms. In more high stake decision making, like examples of medicine, human experts for many, many different reasons. The simplest of all is accountability. They need to stay in the loop. So there will be more focus on augmentation rather than automation. There are different ways to understand this.
There are some real general theories at this point. For example, machines are very good at doing things that are very recurrent, doing things that are very data centric. Do not require much intuition or emotional intelligence, all right? But we are very good at exception handling, which means, when there are things that require judgment calls. For a vast number of people, we are deciding whether they qualify for loans. So this is a data centric type of decision making situation. Machines are quite good at handling masses of applications at the same time. But then when you deny an application, there will be the second decision making. Someone looks at this person’s application, and sometimes you know subjectivity is involved. Other important criteria, like their background, what happened to this person, if this person has in the past, done a bunch of mistakes, but it seems that they are doing well in the past two years. So their credit score is low, but you can put it into context, so that ‘putting things into context’, that requires intuition, that requires emotional intelligence, and I don’t think that part of the workflow can be offloaded to machines.
Ross: So I think a lot about what I could describe as architecture. Humans in the loop is, we keep humans involved in the entire process. But part of his question is, where is the human involved? And as you say, that, of course, is context specific, on the organization, on the type of decision, and so on. But are there any ways in which we can understand different points, or ways in which we bring humans into the loop, in terms of exceptions, as you mentioned, or approvals, or in terms of shaping judgment, or whatever else. What are the ways in which we can architect humans in the loop?
Mohammad: So that the simplest answer that I kind of touched upon earlier is when intuition is needed in decision making. In that article, human AI symbiosis. I said we use these two styles of decision making, intuition based for analytical decision making. Analytical decision making is driven by data, and you can say, AI, artificial intelligence has really concurred that front. Intuition is hard, because mostly happens at the realm of subconscious so anything that requires intuition for decision making, particularly in organization, when we move from the- I’ve done some work on algorithmic management, when algorithms can be used as not necessarily replacement, but aids to managers, when we Move from the lowest level to the highest level of organization, the role of intuition, this is research for management and psychology for many years. This is not something nascent. The role of intuition becomes way more important because intuition is very helpful when you’re concerned with holistic decision making. For example, in organizations when there are multiple stakeholders involved, the decision is not just driven by data, because data often optimizes from the perspective of one of these stakeholders. And in organizations, in most organizational decisionmaking these interests, interests of different stakeholders, is often at conflict. If you maximize one of them, if you help shareholders, your employees will be unhappy, or your customer will be unhappy, right? So that is not a necessarily data centric decision. At the end, it really boils down to judgment call. Where should I strike the balance? When we get to the highest level of strategic decision making, where I use this term earlier, put things into context. AI systems have been able to penetrate some of our contexts. Our context of language, English language, to some extent, is understood by large language model, right? For example, it can understand some of the tacit rules of language. I’ll come back to that term. For non native speakers, understand that some of the worst things that you cannot figure out, and it’s very hard, is when the article in the sentence is needed, ‘A’, and sometimes you don’t need them. In most cases, that is the rules. But sometimes they’re just tacit, you know. When you ask native speakers, why do you do that? What do you put that before this word, their answer is, it just sounds right, right? It’s very useless, but it sounds right. So that’s context, that’s the context of language, that’s the context of English, that is the context of conversation. Yes, these systems have really penetrated some of those processes, but that is a very limited context of human decision making. That’s the limited aspects of our social interaction and organizational context and dynamic.
Ross: You had a recent, very good Business Review article. ‘What will working with AI really require’? In there you lay out this framework for competitive and cooperative skills, both from the human side and the AI side. And I think that’s really powerful. So perhaps you could share what does this actually mean, and can this be put into practice?
Mohammad: A little bit of background. Some people came forward and said, ‘This is the Race Against The Machine’. Some more thoughtful people like Kevin Kelly, they said, ‘No, this is a race with the machine’. In this article, we made a very simple assumption that it’s both. We are racing with and against the machine. So in that dynamic, there are two types of skills that we need to develop, and machines itself. Machines themselves, they need to possess it moving forward, we have to work together. We are partners. I alluded to this earlier. Machines are elevated to the role of partners. I tell students machines of the past, they were support infrastructure. In most cases, they didn’t make decisions. They were decision aids. I don’t think that’s necessarily the case. That’s helped them. These machines are going to help us with augmentation, you know, the argument on decision making, but I imagine one of the major changes in the nature of the workflows of the future is we’ll have machines or AI systems that are co workers or teammates or partners, which is scary, but also interesting, right?
Now, working with these partners requires competitive and cooperative skills. We need to be able to provide something that is competitive. We should give up things that do not make us competitive. Some of our analytical skills of the past, they might not be as useful. You need to understand how certain decisions, certain calculations are done. But I can imagine our education should be transformed, and it will be eventually transformed. One of the major misunderstandings of our time, when I talk to students all the time, and many of them are thinking about their future career, what are the things that they should invest in. I always tell them, this is the bottom line. I’ll tell you the end of the story. It’s continuous learning. You’ve got to learn, right? That is how you make yourself AI proof. What are the things that you got to learn? One of the biggest misunderstandings here is, if you’re close to the machine or to data or to the technical aspects of the system, you are more immune. That is not actually the case, right? Chat GPT, when it was developed, after a while, they basically fired some of the early programmers, because the machine can do some of that work. I’m not saying programmers will go extinct tomorrow. That’s not true, we really need some of these hard technical skills. But that is a misunderstanding, if you’re closely aligned with the machine, you’re developing these machines, you are on the supply side, that gives you an edge. That’s not actually true. So if you look at some of the jobs that are in terms of competitive advantage, some of the jobs that are, I would say, completely AI proof, are preschool teachers, tutors, right? And there are some common grounds in these types of jobs and in that article, we kind of flesh out the commonalities, but I think the one of the major similarities across these types of profession is, as long as we are going to serve humans, the end consumers, some of the major stakeholders in our organizations are humans. You need to have entities, actors, agents, who have emotional and social intelligence. And that is going to stay part of our competitive advantage arsenal. That’s not gonna go away, right? So that is what I call competitive advantage, competitive skills that we got to work on. I tell the students some of the soft skills that are not appreciated enough that they might be more important in your future career. In terms of collaborative or cooperative skills, these guys are going to be our partners. You need to understand how to work with them effectively. So going back to a common example that many people would understand, ChatGPT, you need to understand how you can shape ChatGPT to get what you want to get out of ChatGPT. That metaphor comes with a lot of important dimensions, like number one, understanding ChatGPT is not good at certain types of questions. Understanding these systems, one of the major inherent characteristics is hallucination. I don’t think that’s going to be fixed. That is based on the self learning capacity. The beauty of these systems. And if you want to extinguish self learning, if you want to completely remove hallucination, they won’t be as powerful in self learning. It’s very simple to put it in terms of promptly engineering, but it’s something bigger than that. I would often use this metaphor for understanding how AI may fit into our organizations. In most cases, it’s a very one or two dimensional type of thinker, amazingly smart in some specific areas. But that doesn’t make them good players, team players, because we eventually want to use these technologies as part of our teams and organizations. It requires a lot of integration work. Part of our cooperation with these systems is how we can integrate them into our personal workflow, but also organization. That’s one of the biggest questions that organizations are grappling with, because, to date, systems like chatgpt have been very helpful in increasing personal productivity, but tracing that into organizational impact is a little bit more difficult.
Ross: Yes, yes, the workflow. So from the very start when I was framing humans plus AI, the first frame for me was around humans plus AI workflow and being able to work out what is each good at, and how does that fit together in the system. So to come back to the human competition in terms of, how do we make that healthy, but this comes back to the bigger frame. You’ve got humans, you’ve got AI. In order to build symbiosis, you need to build both sides. And so part of it is the human skills or attitudes, and part of it is the design of the AI. So turning to the AI side for a while, ChatGPT or LLMs of today, we have a particular structure. But how is it that can we design, or what is the next phase of AI so that it is usefully competitive and cooperative with humans?
Mohammad: So I think this is a very difficult thing to do, but we need to really work on the explainability of these systems. One of the major hurdles, particularly in any of these generative AI systems, is the concept of data provenance. Where does this piece of information come from? The power of these systems, if I want to boil it down, the information power of these systems? It is really the synthesis. Like, if you were looking for some of the questions that you’ve been asking from Google, you had to go through pages upon pages to piece it together. These systems remove that step. That is a very, very important strength of these systems for knowledge work. But there is a flip side to it. They need to tell us where they got these pieces of information. And this speaks to the bigger problem of explainability. And again, we know that the other side of explainability is opacity, it’s an inherent problem of self learning and the high level of adaptability that these systems enjoy. Otherwise it becomes difficult to use and integrate these systems seamlessly in our personal life. You can think about all types of problems, accountability. We already see it in our education system, right? The students bringing really interesting analysis. And then when we ask, where does this come from? It’s hard to pin it down right. The concept of data provenance. Data provenance means that the gene of this data. In areas like medicine, it is really important to figure out what page you pulled out this data from. Is it from Reddit or it is from Mayo Clinic, from a reputable medical source, right?
So again, this speaks to explainability. The system really needs to explain how certain decisions have been made. That is one of the major, I would say, cooperative skills that the system can present itself. You can think about all types of you know, in that article, we talk about NLP, like the way that we are talking together. I think these systems have made magnificent progress towards making it natural to communicate. That was the biggest problem of some of the early systems, early as I would say before 2023 it was hard to query these systems because you weren’t sure what to ask. Right now, that process of ideation is actually quite fruitful. We can use these systems to keep asking questions and ideating, and I think that’s a very important part of the way that these guys can augment our creative thinking.
Ross: So one of the most intriguing and interesting aspects of the article is this idea of competition and cooperation. So cooperation is pretty obvious. Yes, we have to cooperate to be able to build something which is more than the sum of its parts. But competition can be either healthy or unhealthy. We can have, of course, healthy competition, where everyone’s stretching and being their best and enjoying the competition. There’s also, of course, unhealthy competition, which can be destructive. So how, from the skills and attitudes from the individuals, and also how we design the systems, can we make this competition as healthy and empowering as possible to people, because otherwise this does have a risk of taking people into this attitude of race against the machine, and I’m competing and I’m losing, and this doesn’t feel good.
Mohammad: So that has been basically the arc of my argument in all other articles I’ve written. Recently, I argued against the Turing test. Some of the benchmarks in computer science, they’re actually not very productive, because the Turing test is about whether the machine can mimic us, can imitate us, right? So that is the idea of competition, and I don’t think that’s a very useful way- that part of the discourse makes people nervous, right? If you go and talk to doctors, sometimes the conversation that more technical folks hold with them comes and trains some of our system, like radiologists annotate some of our data. Those people are smart. Doctors are smart and somehow powerful. They figure what the idea here is. Some of these terminologies come from computer science, like end to end machine learning or last mile problem. End to end means we want to automate the whole process. You guys are helpful to train our algorithms, and you can’t think ‘this can go wrong in many different ways’.
In my work, I’ve been very, very forceful to say this is not organizationally feasible because humans enjoy and present a lot of tacit knowledge, and that tacit knowledge is one of our major source of strength and competitive advantage, so we need to really do some fixing in terms of the language we’re using, like Turing tests. I don’t think it’s very helpful, because the idea here is we need to replicate humans. That’s not going to be a helpful thing. And we are humans, right? But at the end of the day, there are skills that are becoming much more useful in the age of algorithms. Instead, we can do things that are quite unique. I’m old enough to reflect on my education path, we used to memorize a lot of things until very powerful search engines came along, and explicit knowledge became less important. Because look at that generation of our parents, grandparents, sometimes they didn’t have access to information. Now we’ve got some of the most powerful systems in the world to retrieve information, why should I memorize things? AI systems, or even non AI systems are actually quite powerful. The same metaphor can be used here. Some of the things we’ve been teaching our students, some of the cognitive skills that we’ve been giving our students or ourselves, we’ve been equipping ourselves with these skills. I don’t think they’re going to be very useful moving forward, right. Programming will be, I talked about this, programming, data science will be transformed. I cannot pinpoint specific skills, because they really differ in a specific field. That’s what we call domain knowledge. Domain knowledge will be transformed differently. But the idea here is anything that is human centered will be a source of synergy and a source of competitive advantage. I gave you a couple of examples. I think one of the most important ones is tacit knowledge. Things that are path dependent, require practice. Tacit knowledge definition, if I want to make it clear, is things that cannot be easily articulated in words, in writing, and for that reason, might still be external to AI systems, because that’s how they learn, right? They’ve learned some of our tacit knowledge. But one of the most powerful ways that these systems train themselves on things that we’ve been doing, like image processing, writing and things like that, has been ingesting massive amounts of our digital exhaust, digital data, right? Things that we could have made explicit? Through that training, massive brute force training, however you put it, also figuring out some of our tacit right? But that’s limited, because for tacit, sometimes it cannot even be verbalized. It’s a bodily experience. One of the best examples of tacit is how to ride a bike. I can give you some instructions, but you need to go through that experience as a human.
Ross: Yeah, I use the example of surfing as the example of something where information is not the same thing as knowledge, the capability to act. But I think it’s absolutely right in this idea of, as you say, tacit knowledge is what is not made explicit, and the machines have only been trained on the explicit so there’s this big gap there. These are not new ideas. So JCR Licklider wrote ‘manned computer symbiosis 1960’. Of course, it was focused on intelligence augmentation. And I think we kind of seem to have largely lost the plot along the way, in the sense of just focusing always on AI as beating humans. Hopefully now there is a bit more of a movement of human AI symbiosis, or complementarity and so on. What are the most promising research directions? What do we need to be doing in the next few years to really push out the potential of humans and AI working together?
Mohammad: That is a difficult question. In the US, they call it like that’s a very good question when we don’t have a very clear answer. I think we need to grapple with two questions, two basic questions that really guide us through the AI research, the could and should, and they’re both very important when we figure out that symbiotic relationship. Could, which focuses on, is this technologically or organizationally feasible? Because a lot of promises can be made. A lot of important progress can be made in the lab, type of setting, in control, type of environment. But when you bring it to actual organizations, like one of the biggest, most difficult processes in organization, is communicating decision making. If your partner is not able to tell you how they made the decision, how are you going to convince other stakeholders that this is the path moving forward? Even though it is an optimized decision making situation, you need to convince people, different stakeholders, bring them on board, when you make decisions right?
AI systems are not there yet, so they require a very cool collaboration. Some of the technological inherent problems need to be fixed or alleviated, at least to some extent. We need explainability engines, things like that. So that’s the good question, the nexus of technological feasibility and organizational feasibility. Can we do it? But there’s also a very important ‘should’ question, if we can do it, we should always ask the question, should we do it? Should we assign a certain type of decision making to AI systems because they’re very efficient in scaling decisions? A lot of sentiment, unfortunate sentiment in the AI community, particularly when it comes to the business and corporate world is very focused on efficiency goals, which means, how can we make the whole process cheaper and faster? And often a very simple consequence, intended or unintended, is reducing headcounts, and we’ve been through this several- this is not a new problem. We’ve been experiencing this type of approach to information technology, some of the earlier understanding of business process reengineering, and we know that this is not going to work long term. This is a very short sighted, short term perspective. One thing I want to emphasize in the should and could question, and that has been an undertone of a lot of my research, the real power of AI systems, the interesting ones we are seeing today lies in learning.
If you’re using them to just make processes efficient and kick people out, you’re missing the whole point strategic benefits of these systems, which is translating machine learning into organizational learning, to human learning, mutual learning, and then organizational learning, without the mutual learning, and mutual learning is such a powerful ‘should’ normative concept that really help us also with implementation, integration of these systems in organizations, that is how we understand the power of AI, the true power of AI. And that brings us to the goal of effectiveness. You are not just making things efficient. There is a reason that a lot of managers see AI through the lens of efficiency, because it’s quantifiable. You can quantify the dollars that you’ve saved, but that’s not necessarily quality. That’s not necessarily innovation. Innovation is when you can learn as an organization. I think a lot of approaches right now are very focused on just making things efficient, and that doesn’t really help us answer, address these two ‘could‘ and ‘should’ questions.
Ross: That’s fantastic. And I think this frame around building mutual learning, the organization I think is very important and very powerful. So thank you so much for sharing your insights, and also the fact that you focused your energy and attention on what I believe is such an important topic. So thank you for your time, your insights and all the work you’re doing.
Mohammad: I appreciate this conversation. Ross, thank you.
Podcast: Play in new window | Download