“When you can maximize the collective conviction of the group rather than just aggregating their gut reaction with no sense of conviction, you get significantly more accurate answers.”
– Louis Rosenberg
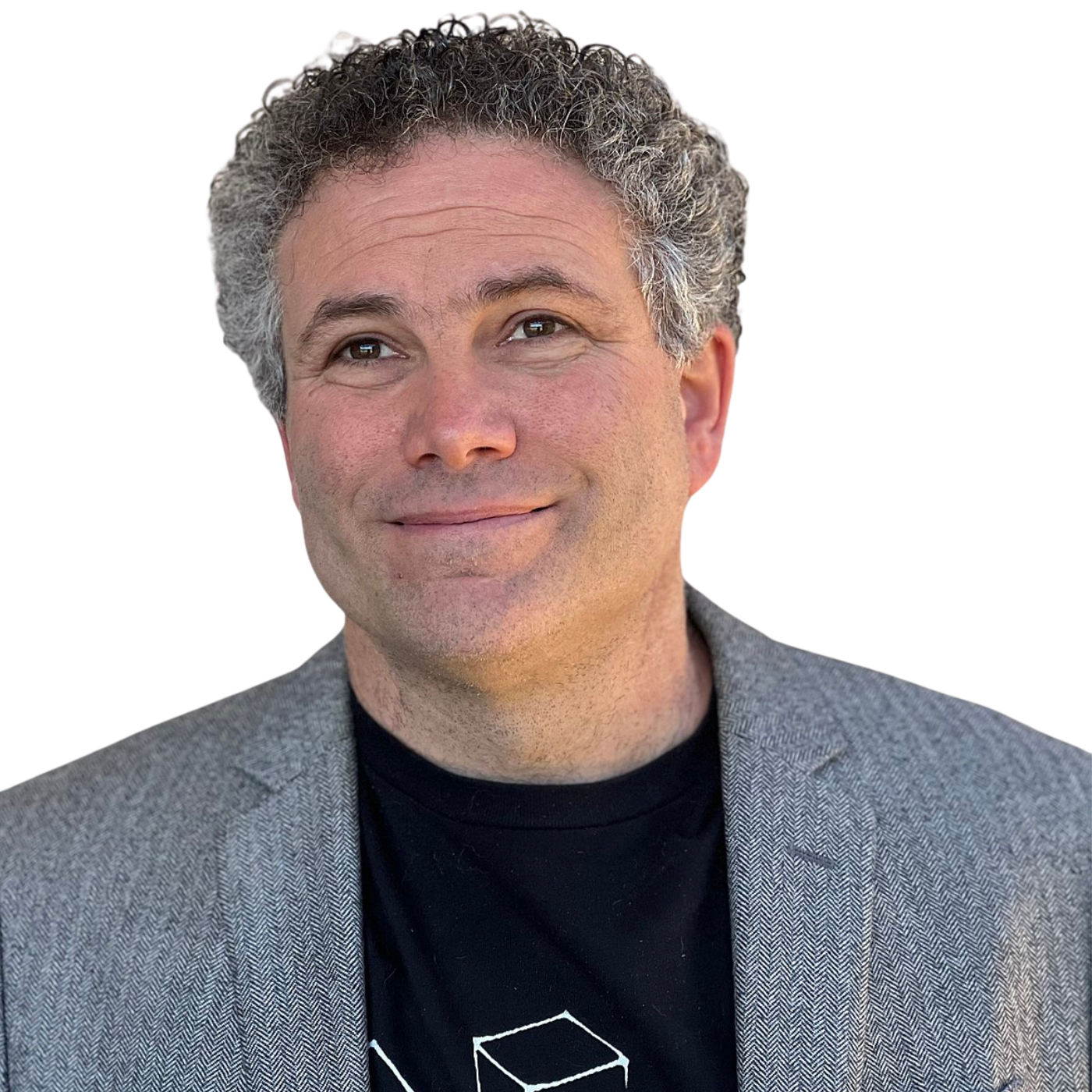
About Louis Rosenberg
Louis Rosenberg is CEO and Chief Scientist of Unanimous A.I., which amplifies the intelligence of networked human groups. He earned his PhD from Stanford and has been awarded over 300 patents for virtual reality, augmented reality, and artificial intelligence technologies. He has founded a number of successful companies including Unanimous AI, Immersion Corporation, Microscribe, and Outland Research. His new book Our Next Reality on the AI-powered Metaverse is out in March 2024.
What you will learn
- Exploring collective intelligence lessons from nature
- Understanding real-time adaptation in natural systems and individual convictions
- Deciphering conviction signals from bee waggle dances
- Unveiling conviction dynamics in collective decision-making
- Exploring the limits of group conversations
- Enhancing group conversations with AI insights
- Envisioning the future of large-scale group conversations
Episode Resources
Transcript
Ross Dawson: Louis, it’s wonderful to have you on the show.
Louis Rosenberg: Yeah, thanks for having me.
Ross: So, swarm intelligence is something that is, seems to be the move to the center of your life. So tell me, what is swarm intelligence? And also why has it captured your imagination?
Louis: Yeah, yeah. So, I spent my whole career looking at technologies that can be used to amplify human abilities. It started out looking at researching technologies like virtual reality, augmented reality, mixed reality back 30 years ago. And about two decades ago, I started kind of transitioning my interest from how do you amplify the abilities of single individuals to how do you amplify abilities of groups. And can you use technology to make groups of people smarter?
Now, there’s an existing research that has been around for 100 years in a field called collective intelligence, where it’s pretty well known that you can take a group of people, ask them a question. The most famous example was about 100 years ago. An experiment by Sir Francis Galton, where he asked 100 people or actually 800 people to estimate the weight of an ox. He took all their individual estimates. I created an aggregation, by statistical aggregation, and the group was smarter. And that birthed this field of collective intelligence. Sometimes people call that the wisdom of crowds. And about a decade ago, it really struck me that the techniques that most people are using in modern times, really haven’t changed that much from 100 years ago.
Most collective intelligence methods are about collecting information from individuals, aggregating and seeing an increase in intelligence, but not a massive increase, but a real increase. And so I did what a lot of people do in a lot of different technology areas, look to nature, and how does nature solve this problem? And it turns out that nature and evolution has been wrestling with this issue of group intelligence for hundreds of millions of years. And evolved methods in a lot of different species that are independently that solve this problem. It’s the reason why birds flock and fish school and bees swarm. They can make better decisions together in groups, than they can as individuals. And it turns out that nature does not do it the way people do.
We don’t survey a bunch of individuals, take the statistical average, and use that as the solution. What nature does is it forms systems, real time systems. And biologists really call those systems swarms. So whether it’s a swarm of bees, or a school of fish, it’s referred to as swarm intelligence, because it’s a real time system. And these natural systems are pretty remarkable. And they are a really good inspiration for how we can make human groups smart. But if you think of a school of fish, for example, 1000s of members, nobody’s in charge, and yet they can make decisions as a unified system. Decisions so quickly that a predator could approach and the whole swarm of fish can evade that predator as a single unit, and yet, there’s nobody in charge.
But it’s not just an evasion of predators. A school of fish makes decisions as a group as a collective, to navigate the ocean and find food and seek waters that are more amenable to their survival. And these species have been around for hundreds of millions of years making decisions like this. And their decisions are smarter as a collective than the individuals could do on their own. And so how do they do it? They do it by forming a system, where each individual in that system has a different set of knowledge. If you think about a school of fish, some fish can only see in one direction, some fish can see in another direction. There’s other fish who can’t even actually see outside the school.
Each organism has a different set of knowledge. It has a different history, or different experiences. Each fish has a different temperament. And so they’re all collecting information, and then they’re all behaving and they have the ability to communicate with each other and fish are pretty amazing. They communicate with each other based on little vibrations in the water around them from their neighboring fish. And so they actually have an organ on the side of their body called a lateral line. And it allows them to detect what the fish right around them are doing? What’s their speed and direction? And so a single fish has this little group of fish that he can detect what it’s doing. It can detect their sentiments. And that fish has its own sentiment. And so it’s having a little negotiation with the fish around them. And then the question is, well, how does that translate into the whole school making a decision? Well, the amazing thing about schools of fish is that each of those little subgroups overlaps with other subgroups.
So one little subgroup is having a conversation, it’s overlapping another subgroup of individuals who are doing this same thing. So information can propagate through the full system really quickly. And that whole fish, that whole group can make decisions. And so whether it’s fish or birds, or bees, especially these groups that function this way, amplify their intelligence. And so about a decade ago, founded a company UNANIMOUS AI just focused on can if this works for birds, and bees and fish, can we let humans do this? Can we connect human groups together in real time systems? Where can the groups push and pull on each other? To answer questions to make predictions to make forecasts? And will it amplify their intelligence and it turns out that it works and it works really well. And we refer to that as artificial swarm intelligence, or swarm AI. And that has been my, like you said, my focus for the last decade. And it continues to amaze us how when groups of people can form these real time systems, they can significantly amplify their group intelligence.
Ross: So first of all, just taking a little bit on the natural phenomenon and then how you sort of replicate that or imitate that. So I remember quite some time ago, people who created algorithms for artificial birds, which basically created flocking behavior. Essentially, where everyone is responding to each other in the way you describe. So, to what degree have the bird or bee or other group behavior been, I suppose, replicated or manifested these algorithms, where we can understand what are the inputs? And what is the behavior of the individuals, which leads to these swarms?
Louis: Yeah, so the key, whether it’s birds, or bees, or fish, that the key thing about how these systems operate, is that each individual doesn’t give a response, like a person on a survey might give a response, let’s go left, like that’s, that’s not. That’s not how these systems work, these natural systems, each organism pulls in a direction in real time and behaves and then reacts in real time to everybody else pulling. And so every organism in the system is adapting its behaviors in real time. It’s in some sense, discovering the strength of their convictions in real time. Based on their pulling in a certain direction. How much do people pull back? If an organism has really strong conviction that they should go a certain direction, they will resist the group that’s trying to pull it back. If they’re ambivalent, they might want to go in a certain direction. And as soon as there’s resistance, they just concede to the group. And so the thing that’s really interesting about a swarm, because it’s this real time system with feedback loops, is that you discover the true level of conviction of each individual. Whereas in a stock survey, or poll or an interview, you don’t really know the level of conviction, and the individual may not even know.
Ross: To talk about conviction when you’re talking about animals. So I mean, yeah, that’s a huge, that’s as far as a human imputation of what it is that these animals are doing. So back to my question, other algorithms that have been used to effectively reflect exactly the behaviors of the swarms?
Louis: So yes, so two things. First, I would say that it’s an underestimate to say that these animals don’t express conviction. Even the simplest of the ones I mentioned are bees, honey bees. They absolutely expressed conviction, in fact, and the algorithms are well known for how honey bees spread conviction. When a group of honeybees makes a decision. What they do, they do something called a waggle dance. Which is to vibrate their body, the magnitude of that vibration is the strength of their conviction. And the direction of that vibration is the strength is the direction that they think that the group should go. And they negotiate based on magnitude and direction of conviction, until they can converge on a solution that the group as a whole can agree upon.
And so, we humans can’t waggle dance. We humans don’t have lateral lines on our body like fish to detect vibrations in the water. And so the first types of systems that we built, created a graphical user interface. Where each person is, each human who could connect from anywhere in the world controls a little graphical icon with their mouse or touchscreen, or just looks like a little magnet. And the magnet is, they use the magnet to pull on the system. And they pull on the system in whatever direction, it’s not a vote. It’s continuous, it’s analog. And so let’s say I put a question up on a screen. And it could be something as simple as you know, who’s going to win the Oscar for Best Picture, and there’s a bunch of different options. And each person controls a magnet, and that magnet has a magnitude and a direction. They have to modulate that in real time. As the swarm starts moving in a direction. I have to chase the swarm with their magnet. And so we emulate the same kind of signal that we see in fish, or even more specifically bees. And now we have trained an AI to look at those signals. To look at the magnitude and direction of how people pull. And use that to infer the strength of their conviction.
Ross: Is conviction a key concept here?
Louis: Conviction is a key concept, because the question is I can ask, I can ask somebody a question. And they can start pulling in, everybody can pull in a different direction. And I have no idea who really cares strongly about that? They all pretty much have equal weight. But I asked you to make a decision. And so you start pulling in a direction. And in fact, if I ask those people to tell me the strength of their conviction, they can’t tell me. And people try to do that on surveys all the time. They’ll say, you know, tell me, who’s gonna win? And on a scale of one to 10, how confident are you? And you can’t do it because eight people aren’t linear in their sense of conviction. My scale and your scale are different. I could say I have a conviction of eight. And you could say you have a conviction of six. And those might actually mean the same thing. But when the group is together in a system, and they’re all behaving, pushing and pulling. And then you have an AI, that’s not looking at what they report, but looking at how they behave. And by mean by how they behave, somebody starts pulling for an option. How long does it take before they realize that they can’t sway the group, and they concede.
The length of time it takes for them to convict us to capitulate to the group is telling us the strength of their conviction. And if you have a multi directional, multi-dimensional problem, if I start pulling for direction, and then I switch to another direction. You know, how long does that take? How aggressively am I? Am I chasing the swarm if it’s moving away from me? So there’s all these subtle behaviors that the AI learns to say. Okay, I understand how a person’s behavior in this system relates to its conviction. And it aggregates everybody’s sentiment in real time, based on those convictions. Which is really the most important value. But it’s also a feedback loop. And this is the subtle point. Which is, I can ask a question. A group of people start pulling in different directions. As soon as the swarm starts moving, everybody starts adjusting their behavior. And so the system keeps getting more and more information about conviction, because it’s, again, it’s not a vote. Everybody’s changing in real time.
And so the question pops up, everybody starts pulling. As soon as the swarm starts moving in a direction, people start adjusting their behavior. The AI is able to define its sense of conviction based on how everybody behaves. And so this one might move in a direction, stop, start moving in another direction, stop and then find the solution. That can combine that basically can maximize. In most cases, the collective conviction of the group. And when you can maximize the collective conviction of the group rather than just aggregating their gut reaction with no sense of conviction. Which is the typical way of doing it. You get significantly more accurate answers. And we’ve partnered with, actually, most major universities to run rigorous tests to see. As this really amplifies intelligence. We ran a test with a set of experiments with researchers at MIT. Looking at financial forecasters. And here the issue was okay, we’ll take groups of financial forecasters. 12 to 20 people and ask them to predict the price of gold, the price of oil and the S&P 500. And do that every week for 25 consecutive weeks. And we’ll ask them to do a survey. We’ll see what their answer is, we’ll ask, we’ll aggregate those surveys to take the most popular answers and see how that does.
And then we’ll have them do it as a real time swarm. And in the published studies, we saw that when they work together as a swarm. We amplify their accuracy by over 25%. 25% more accurate. When we combine your insights together as a swarm. We did a similar study with Stanford University, looking at doctors. And these relatively small groups of doctors making diagnoses. That was four to six doctors, they were going to diagnose X rays. X ray pops up on their screen, all their screens. They could either just take a vote the traditional way. Does this patient have pneumonia or not? In this case, they were asked what’s the probability that this patient has pneumonia. And when they either did it as individuals versus taking a vote. Or an aggregated survey versus doing it together as a swarm. The published study showed that they were over 30% more accurate when they worked together with this real time system and converged on the answer. And in both these cases, when you ask people, why did you interview the participants? They will tell you that they discovered the strength of their conviction in the process. Like the process works in both ways. Like the AI is discovering the conviction of the people. But the people are also discovering how strong they feel. And the only way you really discover how strongly you feel about something is if other people are opposing you if there’s resistance. And that’s the problem with collecting input that’s not in a system. Is that there is no resistance. People are just giving us their gut reaction.
Ross: So that’s obviously, the way you’re describing, is when there is a single set of possible answers.
Louis: Yes.
Ross: And so then you’ve also looked at conversational swarm intelligence, which I suppose is more open ended. So you’d like to sort of dig into that. And you’re reflecting that this is an amplifying cognition podcast. So we want to get very specific around. What are the mechanisms, structures or algorithms? How is it, through conversations, you can bring together better outcomes as a group?
Louis: Yes. So there’s really two principles of human behavior that have been well known. One is collective intelligence. that groups can be smarter than individuals. If you can harness and aggregate their input. The other principle is that human groups are very skilled at reaching good decisions through conversational deliberation. So conversational deliberation is a key human quality. And so, we started from this principle of,we know that conversational deliberation is an important way that groups generate ideas, debate alternatives, surface insights, push back and find solutions as a group. And we know that very large groups can be smarter than small groups. And so the principal test was, okay, well, why can’t we have 500 people have a conversation? Can’t we connect people together now 500 people to have a conversation? It turns out that you can’t do that in a convenient way. Now, can we look at it like a chat room? Like if you wanted to put 500 people in a chat room and have them have a single conversation? Impossible, because the information is flying by like crazy, right? Or 500 people or even 50 people in a zoom call? It’s not really a conversation. It turns out that researchers have found that the ideal size for human conversation is about five to seven people. And above five to seven people. You know, it just degrades quickly because you lose airtime per person. And you also lose the ability for somebody to react to somebody else in real time. If there’s 20 people, now you have to wait so long to react to somebody, you lose your train of thought.
And so conversations fundamentally aren’t scalable. And so to solve this we can look to fish which have this really interesting mechanism. Where they can have thousands of fish and make these rapid decisions. And again, the way they do it is they, because each fish can detect just a small subset of fish around them, and all the subsets are overlapping. By having overlapping subgroups, you can get the benefits of a small group. But the collective benefits of information, it propagates through the full system. And so the next step for us was to say, well, let’s take a group of 100 people or 500, people break them up into overlapping sub groups of conversations. And that will create a really powerful collective intelligence. Turns out that people are terrible at having overlapping conversations. Meaning, if I’m in a conversation with five people, and I overhear another conversation. It will overload my brain, in fact, there’s a name for it, it’s called the Cocktail Party Problem. In a cocktail party, you could have 100 people in a big room. Each broken up into little groups of five or six. And in fact, our brain evolved the opposite capability. Our brain evolved the capability to focus on the five people around us. And deliberately tune out the people further. So we are the opposite of fish. We cannot do this schooling behavior. To amplify our intelligence in a large group. And so when we built this technology called conversational swarm intelligence. By leveraging the power of large language models. And so what we do to solve this problem is we say, Okay, let’s take 100 people, let’s break them up into 20 groups of five people. Which are all really good sizes for local deliberation. Then let’s put an artificial agent into each of those different 20 groups. And that artificial agent is a sixth member of the group who’s not human. And so, we could actually pay attention to two conversations at once. We can’t do that. But it can do that. So we put this artificial agent in these 20 groups. That artificial agent’s job is to listen to the conversation in their little group. Assess the key insights that are being discussed. And then share those key insights with other groups. Pass information through the network. Basically connect the groups together so it can propagate through like it does in a fish school. And all of those little, our agents in all the subgroups are doing that at the same time. And so now you can have 100 people, which is basically 20 groups of five, all overlapping because of their sixth member. Their AI agent member. And you can get the benefits of small deliberations and the benefit of collective intelligence.
Ross: So in this case you got five people, so this is a text based chat? Usually?
Louis: Yes. So right now we’re doing a text based chat. We also can have voice inputs, and people can put voice input into the text based chat. But we’re not quite fast enough to allow full voice, just straight voice chat. But the algorithms, the methods, and the technology really would be the same for voice versus text. But doing it as text based chat.
Ross: So in that case, you’ve got five people who are having a conversation, deliberating a question.
Louis: With an AI agent, who’s participating, so there’s a sixth member.
Ross: So there is an AI agent. Is the agent of each of those groups reflecting the whole of the other 20 groups or some subset of it? So what specifically is it bringing into each conversation?
Louis: So the AI agent in each subgroup is paying attention to the conversation and so in the subgroup. And so there’s 20 different AIs that are paying attention to conversations. And then there is a market, basically a market algorithm. Where the system knows, okay what are the insights that are emerging in all these 20 rooms, and let’s look for which groups have really different insights. Some of them will be thinking the exact same thing, we don’t need to pass that information. But if one group has one set of ideas and it’s very different from another group, we can pick that information to get passed, and then the agent in their room will express it conversationally. It will say, you know, I was watching group six and they think that this movie should win ‘Best Picture’ because of this and this and this. And then the group that hears this can be swayed by that, could respond negatively to it, could just ignore it. But it’s, again, we’re getting. So the one thing, to kind of, tie this all back. The thing that’s interesting about conversations, especially when you have AI agents that are looking at everybody’s sentiments in real time, is that it’s just like a school of fish in a conversation. You’re really looking at people’s behaviors. How are they responding to each other? So I could ask the question, Who’s going to win the Superbowl? And somebody says, Kansas City, and somebody else says, the 49ers. They’re going to speak with different levels of strength and sentiment. They might have different arguments about why the 49ers versus Kansas City. Now, there’s another group having a similar debate, maybe it surfaces a different idea. And so that idea, that insight that surfaced somewhere else can come into this group.
Ross: How’s that? How’s that complement? So how’s that the AI chooses a particular additional perspective to bring to bear into one conversation? What is the aspect of that complimentary conversation so how does it pick that?
Louis: So the fundamental principle of say, a swarm, is to be able to assess people the level of conviction that people have towards all the possible answers, or the possible reasons and solutions. And so if a group is having a local conversation, we will have a sense of their conviction with respect to a subset of the full amount of information. We will have no idea of their strength of their conviction with respect to this other idea that surfaced in another room. So that is a reason to bring that idea in. And so this is really about making sure that we’re mixing information so that we’re getting the strength of their sentiment towards as much of this information that’s available.
Ross: So what is missing from what their conversation is.
Louis: What information is missing? What reasons haven’t they considered and there’s a hierarchy. Meaning, again, if I have 20 groups, we’ve done groups of 400 people, so there could be, you know, 80 groups going on. And so, there’s a lot of overlap between the ideas. And so if there’s one really obscure idea that only surfaced in one room, it has lower priority to beat to go into rooms. But if I’m going to have a small conversation, that’s considered a certain amount of ideas. But there’s some other ideas that have surfaced in 25% of the other rooms, that’s a pretty important one for this room to consider. Because, it’s likely an important idea. And what’s their conviction? Do they resonate with that point? I mean, the point could be when you haven’t considered the weather, that’s going to be on Super Bowl Sunday? And so, what you’re doing is you’re making sure that this information propagates through the full and that you’re, again, what you’re really getting is what is the reaction that people have to information? Either information they haven’t considered before? Do they resist it? Do they? Do they support it? Or if an idea that if they surfaced an idea, which will often happen. Will they concede if somebody pushes back? Will they argue? Will that idea take hold in their little subgroup? Will that propagate to another subgroup? Will it continue to propagate across? And so you can see that some sentiment, some insights, have the ability to propagate. Others will just die out. they don’t have the ability to propagate? And so it’s, ultimately, the ideas and the insight that can propagate across this full group of 400 people and generate a strong support group is where the collective conviction emerges. And what we see is that those answers, those solutions, end up being either more accurate if we’ve run a variety of tests. Either is more accurate, if there’s an objective answer.
Ross: Okay, well, let’s just run that out. So where do we go from here? So in the next 3, 5, 10 years, where are the pathways to be able to get this to the potential to bring together collective intelligence?
Louis: Yeah. So the experiments that we’ve been doing, and we’ve been working with researchers at Carnegie Mellon and some other places to conduct rigorous experiments. We’ve found that it’s very promising. When we bring groups together in this strange structure, they like it. They understand it. Which is important because they won’t do it unless they can actually feel like a natural way to interact. They turned out they contribute more than 50% more content conversationally than if you just put them all together in one large group. And we see their intelligence increase. One of the most interesting studies that we just did.
Ross: So yeah, so just saying, five years from now, where are we going to be? And what what’s the specific things that are going to have been done before by them to advance the field?
Louis: Right, so five years from now, I think we’ll be able to bring together really large groups. So right now, we’ve done 3 to 400 people. We could bring together 10,000 people in a single conversation. And we could harness their collective insights. And allow them to quickly converge on solutions that are significantly smarter than any individual could, which would generate on their own. And those insights will either again, there could be objective solutions. Where those insights are more accurate or more insightful or their subjective solutions. Let’s say you just want to know, what do you want to know, what directions, what ideas, what sentiments, does the group support the most? Which is the idea that will resonate most with this population? We surface these subjective insights, or we surface these objective solutions. And because it’s conversational, it’s completely open ended. You can start with no idea of what the answers are, and just watch groups and brainstorm ideas, debate ideas, converge on solutions that again, combine the benefits of large groups, the collective intelligence of large groups with the deliberative power of small focus conversations.
Ross: So essentially applying the current approaches and structures. You know, further, rather than you seeing any additional advances in these structures or algorithms?
Louis: I think we will, we will see more and more advances just in how we architect the information passing around. How the artificial agents behave to extract behaviors from the participants? This is a new technology, there’s, I think, lots of room for improvement. What we’re excited about is the basic structure and architecture. It works with humans, meaning it’s something you could tell it could work on paper, it should work, but people, people can actually do it, if they feel comfortable doing it. They actually like participating in this way. And the big thing that we’re working on is how to allow the AI agent and the humans to work better and better together. There’s a lot of interesting open questions about the etiquette between the AI agent and the humans in that conversation. To maximize the
Ross: Yeah, I can imagine that will probably certainly be improved. So, Louis, where can people find out more about your work?
Louis: Yeah, so our company is called UNANIMOUS AI, which is just a unanimous.ai. And yeah, we’ve been working on this idea of artificial swarm intelligence for almost a decade. Conversational swarm intelligence. We’ve only been testing it for about a year and it’s very, very promising and moving very, very quickly. But we publish, we put links to our academic papers on the website and lots of other information.
Ross: Excellent. All right. Thanks. Very interesting work. Thanks for all of your contributions to collective intelligence.
Podcast: Play in new window | Download