“We humans often tend to be very restricted—even when we are world champions in a game. And I’m very optimistic that AI will surprise us, with very different ways of solving complex problems—and we can make use of that.”
– Jennifer Haase
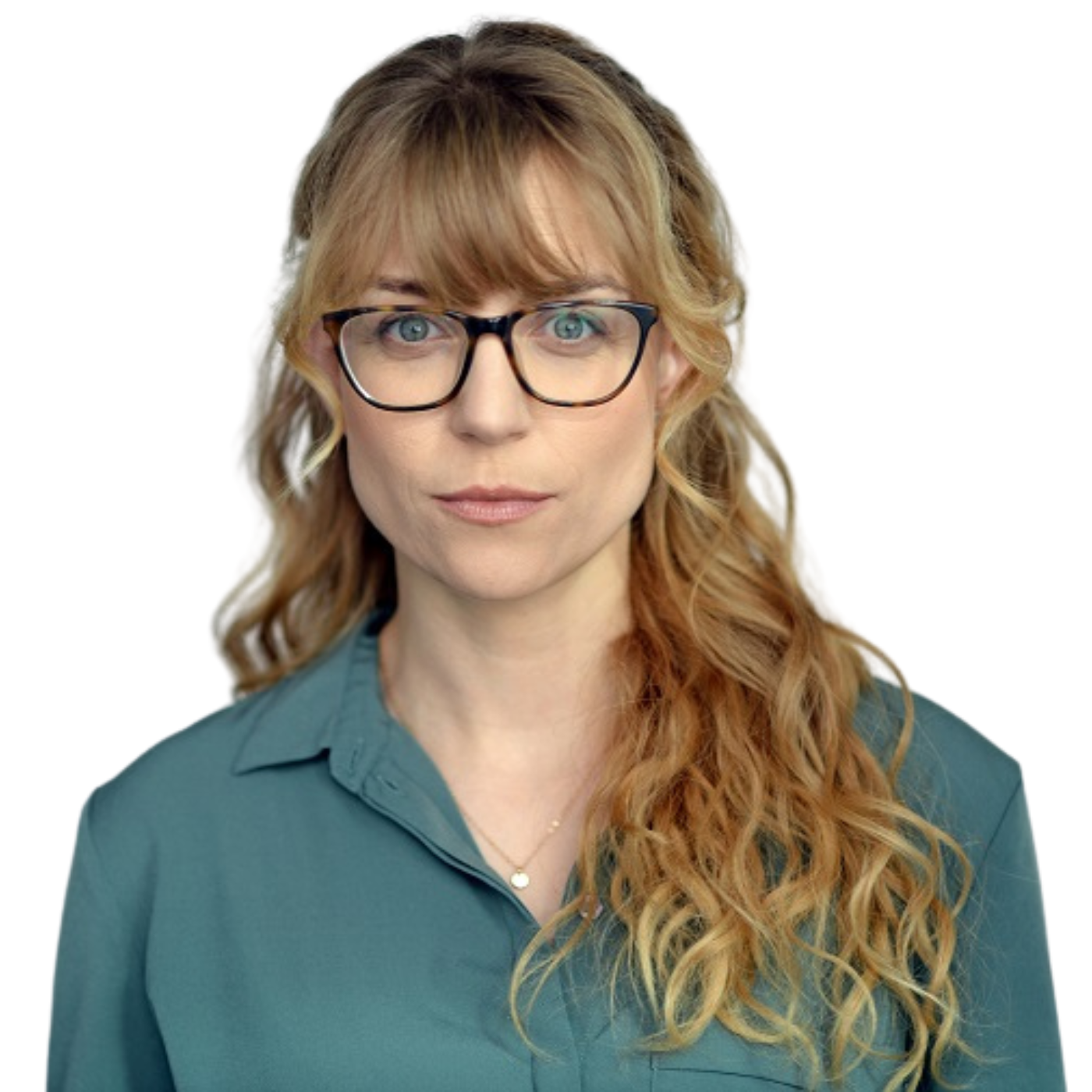
About Jennifer Haase
Dr. Jennifer Haase is a researcher at the Weizenbaum Institute, and lecturer at Humboldt University and University of the Arts Berlin. Her work focuses on the intersection of creativity, Artificial Intelligence, and automation, including AI for enhancing creative processes. She was named as one the 100 most important minds in Berlin science.
What you will learn
- Stumbling into creativity through psychology and tech
- Redefining creativity in the age of AI
- The rise of co-creation between humans and machines
- How divergent and reverse thinking fuel innovation
- Designing AI tools that adapt to human thought
- Balancing human motivation with machine efficiency
- Challenging assumptions with AI’s unconventional solutions
Episode Resources
Websites & Platforms
Concepts & Technical Terms
Transcript
Ross Dawson: Jennifer, it’s a delight to have you on the show.
Jennifer Haase: Thanks for inviting me.
Ross: So you are diving deep, deep, deep into AI and human co-creativity. So just to hear—just back a little bit—sort of how you’ve embarked on this journey. I mean, love to—we can fill in more about what you’re doing now. But how did you come to be on this journey?
Jennifer: I would say overall, it was me stumbling into tech more and more and more. So I started with creativity.
My background is in psychology, and I learned about the concept of creativity in my Bachelor studies, and I got so confused, because what I was taught was nothing like what I thought creativity was—or how it felt to me.
It took me years to understand that there are a bunch of different theories, and it was just one that we were taught. But that was the spark of the curiosity for me to try to understand this concept of creativity. And I did it for years.
Then, by pure luck, I started a PhD in Business Informatics, which is somewhat technical. The lens of how I looked at creativity shifted from the psychological perspective more into the technical realm, and I looked at business processes and how they are advanced by general technology—basic software, basically.
Then I morphed—also, by sheer luck—I morphed into computer science from a research perspective. And that coincided with ChatGPT coming around, and this huge LLM boom happened two, three years ago.
And since then, I’m deeply in there. I just fell, fell in this rabbit hole.
Ross: Yeah, well, it’s one of the most marvelous things. So the very first use case for most people, when they first use ChatGPT, is: write a poem in the style of whatever, or essentially creative tasks. And pretty decently does those to start off—until you sort of started to see the limitations at the time.
Jennifer: Yeah, and I think it did so much. It’s so many different perspectives.
I think we—as I said, I studied creativity for quite a while—but it was never as big of a deal, let’s say. It was just one concept of many. But since AI came around, I think it really threatened, to some part, what we understood about creativity, because it was always thought of as this pinnacle of humanness—right next to ethics.
And I think intelligence had its bumps two or three decades ago, but for creativity, it was rather new. So the debate started of what it really means to be creative.
I think a lot of people also try to make it even bigger than it is. But I think it is as simple as—a lot about creativity is, for example, in terms of poets—poetry is language understanding, right? And so LLMs are really good at it. And it’s just the case. It’s fine.
I think we can still live happy lives as humans, although technology takes a lot over.
Ross: Yes. So humans are creative in all sorts of dimensions. AI has complementary—let’s say, also different—capabilities in creativity.
And in some of your research, you have pointed to different levels of how AI is supporting us in various guises—through being a tool and assistant, through to what you described as the co-creation. So what does that look like?
What are some of the manifestations of human-AI co-creativity, which implies peers with different, complementary capabilities?
Jennifer: Yeah, I think the easiest way to look at it is if you imagine working creatively with another person who is really competent—but the person is a technical version of it, and usually we call that AI, right? Or generative AI these days.
So the idea is that you can work with a technical tool from an eye-to-eye level. Really, the tool would have a—well, now we’re getting into the realm of using psychological terms, right—but the tool would have a decent enough understanding so it would appear competent in the field that you want to create.
I think the biggest difference we see to most common tools that we have right now—which I would argue are not on this level yet—tools like ChatGPT and others, they follow your lead, right? If you type in something, they will answer, sometimes more or less creatively.
But you can take that as inspiration for your own creativity and your own creative process. And that really holds big potential. It’s great.
But what we are envisioning—and seeing in some parts already happening in research—I think this is the direction we’re going to and really want to achieve more: that we have tools that can also come up with ideas, or important input for the creative problem.
Not—when I say on their own—I don’t mean that they are, I don’t know, entities that just do. But they contribute a significant, or really a significant part of the creative process.
Ross: So, I mean, we’ll come back a little bit to the distinctions between how AI creativity contrasts to human creativity. But just thinking about this co-creative process—from your research or other research that you’re aware of—what are the success factors? What are the things which mean that that co-creation process is more likely to be fruitful than not?
Jennifer: I think it starts really with competence. And I think this is something, in general, we see that generative AI just became extremely good at, right?
They know, so to speak, a lot and tailor a lot of knowledge, and that is very, very helpful—because we need broad associations, coming from mostly different fields, and connect that to come up with something we consider new enough to call it creative.
That is a benefit that is beyond human capabilities, right? What we see right now those tools are doing—that is one part. But that is not all.
What you also need is the spark of: why would something need to be connected? And I think that is especially where raising the creative questions, coming up with the goal that you want to achieve something too, is still the human part.
But—it doesn’t need to be. That’s all I’m saying. But still, it is.
Ross: So, I mean, there are some—very crude workflows, as in, you get AI to ideate, then humans select from those, and then they add other ideas, or you get humans and then AI sort of combines, recombines.
Are there any particular sequences or flows that seem to be more effective?
Jennifer: It’s interesting. I think this is also an interesting question for human creative work alone, even without technology—like, how do you achieve the good stuff, right?
And I think what you just described, for me, would be kind of like a traditional way of: oh, I have a need, or I have a want—like, I want to create something, or I want to solve something, or I need a solution for a certain problem. And I describe that, and I iterate a best solution, right?
This is part of what we call the divergent thinking process. And then, at a certain point, you choose a specific solution—so you converge.
But I think where we have mostly the more interesting creative output—for humans and now also especially with AI—is that you kind of reverse the process. So let’s assume you have a solution and you need to find issues for it.
For example, you have an invention. I think—yeah, I think it was that there’s this story told about the Post-its, you know, the yellow Post-its. So they were kind of invented because someone came up with glue that does not stick at all—like, really bad glue.
And they had this as the final product. Now it’s like, “Okay, where can you make use of it?” And then they came up with, “Oh, maybe, if you put it on paper, you can come up with these sticky notes that just glue enough.” So they hold on surfaces, but they don’t stick forever, so you can easily erase them.
They’re very practical in our brainstorming work, for example.
And this kind of reverse thinking process—it’s much more random. And for many people, it’s much more difficult to open up to all the possibilities that can be.
What I’ve seen is that if you try to poke LLMs with such very diverse, open questions, it can be very interesting what kind of comes out there.
Ross: Though, to your point, I mean, this is the way—the human frames, the AI can respond. But the human needs to frame—as in, “Here is a solution. What are ways to be able to apply?”
Jennifer: And all the examples—like, what I’m thinking of right now—is what is working with the tools that we have with LLMs.
And I think what you were asking me before about the fourth level that we described with this co-creation—these are tools that work a bit differently. These are tools that, for now, mostly exist in research because you still need a high level of computational knowledge.
So, the work that I did—the colleagues that I work with—are from computer science or mathematicians who program tools that know some rules of the game, or some—let’s call them—boundary conditions of our creative problem that we are dealing with.
And then the magic—or the black box magic—of AI is happening. And something comes out. And sometimes we don’t really understand what was going on there. We just see the results.
And then, with such results, we can iterate. Or maybe something goes in the direction as we assume could be part of the solution.
So it becomes this iterative process between an LLM or AI tool doing something, we’re seeing the results, saying yes or no, nudging it into different directions, and so, overall, coming up with a potentially proper solution.
This is—at least in the examples that we see.
And if you have such a process and look over it, like what was happening, often what we see is that LLMs or AI tools in general—with their, let’s call it, broad knowledge, or the very intense, broad computational capacities that they have—they do stuff differently than we as humans tend to do stuff.
And this is where it becomes interesting, right? Because now we are not bounded in this common way of thinking and finding associations, or iterating smaller solutions.
Now we have this interesting artificial entity that finds very different ways of solving complex problems—and we can make use of that.
Of course, we can learn from that.
Ross: Absolutely. And I think you’ve pointed to some examples in your papers. I mean—other, sort of, I suppose we’ve been quite conceptual—so examples that you can give of either what people have done, or projects you’ve been involved with, or just types of challenges?
Jennifer: I think—to explain the mechanism that I’m talking about—I think the first creative, artificial example, like the real, considered properly creative example, was when AlphaGo, the program developed to play Go—the game similar to, or somewhat similar to, chess but not chess—when this tool was able to come up with moves, like play moves, which were very uncommon.
Still within the realm of possibilities, but very, very uncommon to how humans used to play.
And so, I think what this new was back in 2016, right? When this happened—when DeepMind, from Google, built this tool and kind of revolutionized AI research.
What it showed us is exactly this mechanism of these tools. Although they are still within the realm of possibilities—still within what we consider the rules, right, of the game—it showed some moves which were totally uncommon and surprising.
And I think this shows us that we humans often tend to be very restricted. Even when we are world champions in a game, we are still restricted to what we commonly do—what is considered a good rule of thumb for success.
And I’m very optimistic that AI will surprise us, like in this direction—with this mechanism—quite a lot in the future.
Ross: Yeah, and certainly, related to what you’re describing, some similar algorithms have been applied to drug discovery and so on.
Part of it is the number-crunching, machine learning piece, but part of it is also being able to find novel ways of folding proteins or other combinations which humans might not have envisaged.
Jennifer: Yeah, exactly. And exactly—it’s in part because these machines are just so much more advanced in how much, or how many, information they can hold and combine.
This is, in part, purely computational. It’s a bit unfair to compare that to our limited brains. But it’s not just that. It’s not just pure information, right?
It’s also how this information is worked upon, or the processes—how information is combined, etc. So I think there are different levels of how these machines can advance our thinking.
Ross: So one of the themes you’ve written about is designing for synergies—how we can design so that we are able to be complementary, as opposed to just delegating or substituting with AI.
So what are those design factors, or design patterns, or mentalities we need?
Jennifer: Well, I will propose, first up—I think it’s extremely complicated. Not complicated, but it will become a huge issue.
Because, let’s say, if technology becomes so good—and we see that right now already with LLMs like ChatGPT—it’s so easy for us. And I mean that in a very neutral way. But lazy humans as we are—I think we are inherently lazy—it’s really tough for us to keep motivated to think on our own, to some degree at least, and not have all the processes overtaken by AI.
So, saying that, I think the most essential, most important part whenever we are working with LLMs is: we have to keep our motivation in the loop—and our thinking to some degree in the loop—within the process.
And so, we need a design which engages us as humans.
I think it’s easily seen right now with LLMs. When you need the first step in—like typing some kind of prompt, or even in a conversation—you have to initiate it, right? You have to come up with, maybe even, your creative task at first.
And I think this will always be true, because we humans control technology by developing it, right?
But even when you’re more on the user end—forcing us to be in the loop, and thinking it through, and controlling the output, etc.—is one part.
But I think what it also needs, especially for the synergy, is for the technology to adapt to us—to serve us, so to speak.
And I think this is an aspect that is a little bit underdeveloped right now. What do I mean by that?
I want a tool that serves me in my thinking. It should be competent enough that I perceive it as a buddy—eye to eye. That is the vision that I have.
But I still always want the control. And I want it to adapt to me, and that I don’t have to adapt too much to the tool.
Right now, we’re mostly just provided with tools that we need to learn how to deal with. We need to understand how prompting works, etc., etc. And I want that reversed.
I want tools which are competent enough to understand, “Okay, this is Jenny. She is socialized in this way. She usually speaks German,”—whatever kind of information would be important to get me involved and understand me better.
I think this is the vision for synergy that I’m thinking of.
Ross: No, I really like that. The idea of designing for engagement, because instead of saying, yeah, why is it going to make us want to be engaged and continue the process and want to want to be involved, as opposed to doing the hard work of telling the—keep on telling the AI to do stuff.
Jennifer: Yes, and also sometimes—I mean, I work a lot with ChatGPT and other similar tools—and sometimes I’m like, I found myself, I hope I don’t spoil too much, but sometimes I find myself copy-pasting too much because there’s nothing left for me to do.
And to some degree, it can happen that the tools are too good, right? Because they are meant to create the output as the output, but they are not meant to be part of this iterative thinking process.
I think you can design it much better and easier to go hand in hand with what I’m thinking and what I want to advance. Maybe.
Ross: Yeah, yes, otherwise the onus is on the human to do it all. So in one of your papers, you identify—you used a number of the different models, and I believe you found that GPT-4 was the best for a variety of ideation tasks.
But you’ve also done some more recent research. I’d love to hear about strengths, weaknesses, or different domains in which the different models are good, or—
Jennifer: Yeah, that’s quite interesting, right? Because—okay, so going back to the start of the big—let’s call it the big boom of LLMs, right?
I think it was early ’23, right, when ChatGPT came around. End of ’22. Okay, so it took a while when it reached Germany—it was for us. No, just joking.
But okay, so around this time, what we found was intense debates arguing that, although these tools are generative, they cannot be creative. And that was the stance held tightest—maybe especially from creativity researchers and mostly psychologists, right?
As I mentioned before, it’s a little bit of this fear that too much is taken over by technology. I think that is a strong contributor—even among researchers.
So what we went out to do is—we basically wanted to ask LLMs the same creativity measures as we would do for humans. Like, when you want to know if a person holds potential for creative thinking, you ask them creative questions, and they have to perform—if they want to.
And that’s exactly what we did with LLMs.
Back in the day, we did it with the LLMs that were easily reachable and free in the market—like ChatGPT. And now, we really redid it with the current LLMs, with the current versions.
And—I don’t know if you’ve seen that—but most LLMs are advertised, when the new versions come out, usually they are advertised with: they are more competent, and they are more creative.
And so we questioned that. Is that really true? Is ChatGPT 4.5, for example—the current version—is it more creative than 3.5 back in the day?
And what we find is—it’s so messy, actually. Because for some tools, yes, they are a bit more creative than they used to be two years ago. But the picture is really not clear.
You cannot really tell or say or argue that the current versions we are having are more creative than two years ago—or even more creative than humans.
It’s been interesting. We’re not really sure why. But all we can say is that, on average, these tools are as good at coming up with everyday-like uses or everyday-like ideas for everyday problems.
They are, on average, as good as humans—random humans picked from surveys.
And I think that is good news, right? Because LLMs are easier to ask than random humans most of the time.
But the promise that they become more and more creative with every new release, in our perspective, does not hold up.
So that is the bigger, bigger picture. Let’s start there.
Ross: So that’s very interesting. So this is using some of the classic psychological creativity tests. And so you’re applying what has for a long time been used for assessing creativity in humans, and simply applying exactly the same test to LLMs?
Jennifer: And to be fair, within the creativity research community, we agree that those tests are not good. Okay, they’re really pragmatic. We totally agree on that, so we do not have to fight for this point.
But it’s commonly what we use to assess human potential for creative thinking—or even more concise, for divergent thinking—which is only one important, but just one aspect, of the whole creative journey, let’s say.
And it basically just asks how good you are, on the spot, at coming up with alternative uses for everyday products like a shoe or toothbrush or newspaper.
And of course, you can come up with obvious uses. But then there are the creative ones, which are not so easy to think of, right? And LLMs are good at that.
They will deliver a lot of ideas, and quite a few of those are considered original compared to human answers.
We also now used another test, which is a little bit more arbitrary even, but it proved to be somewhat of a good predictor for creative performance overall. And that is: you are asked to come up with 10 words which are as different from each other as possible.
So very pragmatic again.
And these LLMs—as they, you know, know one thing, and that is language—are, again, quite good at that on average.
But it’s not that you see that they are above average, or that a specific LLM would be above average. We see some variety, but the picture, I would say, is not too clear.
And also, to mention—which was a little bit surprising to us, actually—is that those LLMs, we asked them several times, like, a lot of times, and the variance in terms of originality—the variance is quite huge.
So if you ask an LLM like ChatGPT for creative ideas, sometimes you can have quite a creative output, and sometimes it’s just average.
Ross: So you did say that you’re comparing them to random humans. So does that mean that generally perceived-to-be-creative humans are significantly outperforming the LLMs on these tasks?
Jennifer: Yeah, yeah. So, but the thing is, there is usually no creative human per se. So there’s nothing about a human that makes a human per se creative.
We tend to differ a little bit on how well we perform on such tasks. Yes, we do differ in our mental flexibility, let’s say. But a creative individual is usually an individual which found a very good fit between their thinking, their experience, and the kind of creative task they’re doing.
And just think about it, because this creativity can be found in all sorts of domains, right? And people can be good or less good in those domains, and that correlates highly with the creativity.
So when we ask about the general, like, the ideas for everyday tasks, there is not really the creative individual, right?
They are motivated individuals, which makes a huge difference for creativity measures. If you’re motivated and engaged, that is something we take as granted.
For LLMs, I guess if you compare them, the motivation is there.
But what we see in terms of the best answers—the most original answers in our data sets—most of the time, not all, but most of the time, come from humans.
Ross: Very interesting. So, this is the Amplifying Cognition podcast, so I want to sort of round up by asking: all right, so what’s the state of the nation or state of the world, and where we are moving in terms of being able to amplify and augment human cognition, human creativity?
So I suppose that could be either just, improving human creativity, or collaborating, or, you know, this co-creativity.
Jennifer: I think the potential for significant improvements and amplifications has never been better. But I think at the same time as I’m saying that, I think the risks have never been higher.
And that is because, as I said, we are lazy people. That’s just what humanist means—and that is fine—but it also means that we have a great risk of using these technologies not for us, but being used by them, basically, right?
So we can use ChatGPT and other tools to do the task for us, or we can use them to do the task more efficiently and better with them.
I think this difference can be very gradual, very minor, but it makes the whole difference between success and big dependencies—and potentially failure.
Ross: Yeah, and I think you make a point—which I often also do—which is over-reliance is the biggest risk of all, potentially.
Where, if we start to just sort of say, “This is good, I’ll let the AI do the task, or the creativity, or whatever,” it’s dangerous on so many levels.
Jennifer: Because it does good enough most of the time, right?
Technology became so good for many tasks—not all, but many tasks—that it does it good enough. And I think that is exactly where we have the potential to become so much better, right?
Because if you now take the time and effort that we usually would put into the task itself, we could just improve on all levels.
And that is the potential I’m talking about. I think a lot is to be advanced, and a lot is to be gained—if we play it right.
Ross: And so, what’s on your personal research agenda now?
Jennifer: Oh, I fell into this agentic LLM hole.
Yeah, no, no—it’s not just looking at individual LLMs, but to chain them and combine them into bigger, more complex systems to have—or work on—bigger and complex issues, mostly creative problems, and see where the thinking of me and the tool, yeah, excels, basically, right?
And where do I, as a human, have to step in to fine-tune specific bits and pieces and really find the limits of this technology if you scale it up?
That’s my agenda right now.
Ross: I’m very much looking forward to reading the research as you publish it.
Jennifer: Thank you.
Ross: Is there anywhere people can go to find out more about your work?
Jennifer: Yeah, I collect everything on jenniferhaase.com. That’s my web page. It’s hugely up to date there, and you can find talks and papers.
Ross: Fabulous. Love the work you’re doing. Jennifer, thanks so much for being on the show and sharing.
Jennifer: Thank you very much. It was—yeah, I love to talk about that, so thanks for inviting me.
Podcast: Play in new window | Download