“In collective reasoning, one of the fundamental hurdles is coming up with a shared understanding of what we’re trying to do, and where we’re trying to go. “
– Anita Williams Woolley
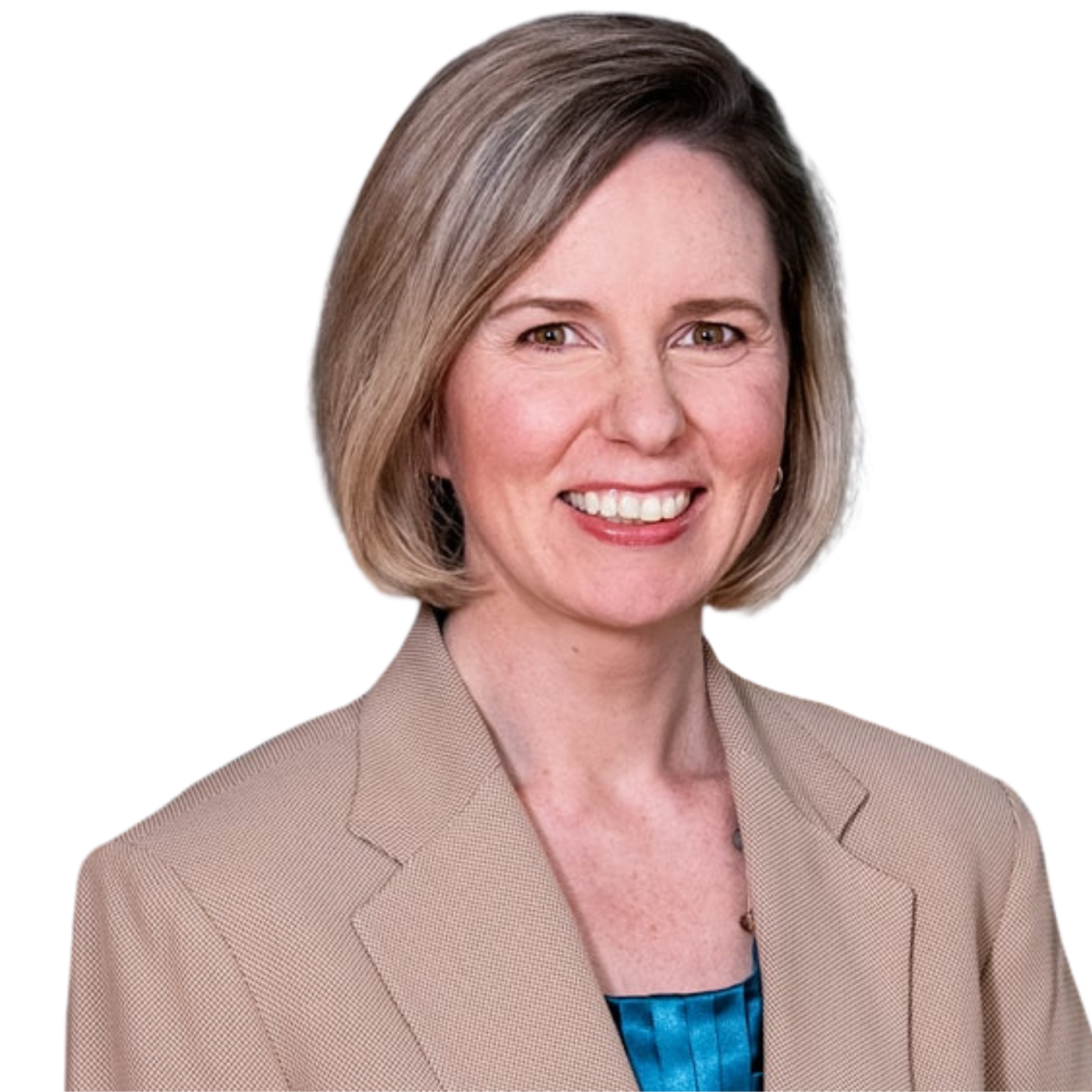
About Anita Williams Woolley
Anita Williams Woolley is the Associate Dean of Research and Professor of Organizational Behavior at Carnegie Mellon University’s Tepper School of Business. She received her doctorate from Harvard University, with subsequent research including seminal work on collective intelligence in teams, first published in Science. Her current work focuses on collective intelligence in human-computer collaboration, with projects funded by DARPA and the NSF, focusing on how AI enhances synchronous and asynchronous collaboration in distributed teams.
University Profile: Anita Williams Woolley
LinkedIn: Anita Williams Woolley
Google Scholar: Anita Williams Woolley
ResearchGate: Anita Williams Woolley
X: @awoolley95
What you will learn
- Exploring the concept of collective intelligence
- The difference between individual and collective intelligence
- How collective memory, attention, and reasoning work
- The impact of gender on collective intelligence
- The role of AI in facilitating human collaboration
- Integrating AI as a teammate in group settings
- Future possibilities for human-AI collaboration in problem-solving
Episode Resources
Transcript
Ross Dawson: Anita, it’s wonderful to have you on the show.
Anita Williams Woolley: Thanks for having me.
Ross: So your work is absolutely fascinating. So I’d like to dive in as much as we can, in the time that we have. Much of your work is centered around collective intelligence and I’d love to just pull back to get that framing of collective intelligence relative to human intelligence. So we have some idea of artificial intelligence, which is emerging. So where does collective intelligence fit in that?
Anita: Yeah, well, it is. There are a lot of uses of the word intelligence. So it’s good to get some clarity. I guess starting with the notion of individual general intelligence, which is the thing that’s most familiar to most people, it’s this notion that individuals have this underlying capability to perform across multiple domains. And that’s what’s been shown empirically, anyway.
So individual intelligence is a concept most people are familiar with. It refers to this. Well, when we’re talking about general human intelligence, it’s a general underlying ability for people to perform across many domains. And empirically, it’s been shown that measures of individual intelligence predict somebody’s performance over time. So it’s a relatively stable attribute. For a long time, when we thought about intelligence and teams, we thought about it in terms of the total intelligence of the individual members combined, the aggregate intelligence.
But in our work, we kind of challenged that notion, by conducting studies that showed that there were some attributes of the collective the way the individuals coordinated their inputs, and worked together and amplified each other’s inputs. That was not directly predictable from simply knowing the intelligence of the individual members. And so collective intelligence is the ability of a group to solve a wide range of problems. And it’s something that also seems to be a stable collective ability. Now, of course, in teams and groups, you can change the individual members, and other things can happen that might alter the collective intelligence more readily than you could with an individual in terms of individual intelligence, but we do see that it is fairly stable over time and enables this, you know, greater capability. In some cases, at least, collective intelligence can be higher when you have a higher collective intelligence than a group that is more capable of solving more complex problems.
And then, yeah, I guess you also asked about artificial intelligence, right? And so when computer scientists start working on ways to endow a machine with intelligence, what they are essentially doing is providing it with the ability to reason to take in information to perceive things, to kind of identify goals and priorities and to reason and to change and adapt based on information that it receives, which is something humans do quite naturally, so we don’t really think about it. But without artificial intelligence, a machine only does what it’s programmed to do. And that’s it. And so it can do a lot of things that humans can’t do even then, usually computations, or some variant of that. But with artificial intelligence, suddenly, a computer can make decisions and draw conclusions that are difficult for even their programmers to understand the basis of so that’s where things get really interesting.
Ross: So we’ll probably come back to that. So yeah, we’re amplifying cognition, we’re all about understanding the nature of cognition. And so one of the, I think, fascinating areas of your work is looking at memory, attention, and reasoning as fundamental elements of cognition. But being able to look at that not just as individual memory, or attention reading, but as collective memory, tensioning, and reasoning. So I’d love to just understand how this looks, what is collective memory, collective attention, and collective reasoning, and how do those play out into I suppose this aggregate cognition?
Anita: Yeah, I think it is an important question because again, just like we can intervene to improve collective intelligence, perhaps more readily, we may also…well, we know we can intervene to improve collective cognition and so Well, as you mentioned, memory and attention and reasoning are three essential functions that any intelligence system needs to satisfy needs to perform. Whether we’re talking about humans or computers or human and computer systems or other sorts of biological systems. And so in when we talk about them and collectives, it’s something that I say collectives because we often are thinking about the superset of humans and human and computer collaborations.
But when we think about collective cognition, it’s something that has been researched in parallel with the work on collective intelligence for a couple of decades now, probably the longest-standing area of research is on collective memory or one specific construct, there is transactive memory systems. And this is something that researchers, some of my colleagues at Carnegie Mellon, Linda Argote being a notable example, have done a lot of work on. And it’s this notion that if you have a strong collective memory, a good transactive memory system, that the group can, can sort of remember, can use much more information and total than they could if they didn’t have this well-constructed transactive memory system. And so, in essence, over time, individuals might specialize in what they’re remembering. The group has a queue so that they know, who is sort of retaining different pieces of information so that they know they don’t need to retain it themselves, but they know where to go and get it. And so as this system forms, again, as I mentioned, the total capacity of information that it can manage, can actually grow considerably.
And so similarly, with transactive attention, we also have a total attentional capacity if we’re working with a group on a problem. And so being able to coordinate, sort of where each person’s focus is when our focus needs to come together. And we need to work synchronously when we should be dividing our attention across different tasks. And again, knowing who’s focusing on what, so that you don’t have redundancies, or gaps, or things like that, and that you can adapt as the situation changes.
Collective reasoning is an interesting one. And it’s an area that actually has a lot of work, but it’s been happening in different pockets. And so part of what we’ve been doing in our work on this topic is really to kind of pull together these different threads and use that as a basis for further understanding how this plays out. But collective reasoning at the foundation is really about goal setting. Because the foundation of a reasoning system is identifying when there’s a gap between a desired state and a current state, and conceptualizing what needs to be done to close that gap. And so in collective reasoning, one of the fundamental hurdles is coming up with a shared understanding of what we’re trying to do, and where we’re trying to go. And then, you know, what are the priorities in terms of how we’re gonna get there, what the interim goals might be, or maybe there are multiple goals that we’re pursuing simultaneously. And so that’s the kind of foundation of it and then adapting those, of course, over time to make sure that members’ personal motivations are fulfilled, because if members aren’t aligned on the goals, they’re probably going to decide that their time is more valuably spent elsewhere like they could go elsewhere, and put in their effort towards something else that they find more rewarding or fulfilling. And so the foundation of collective reasoning is that kind of goal-setting and alignment process.
Ross: Fabulous. I think the point around collective reasoning is, in a way, to a point role allocation, which can take us to sort of human and AI and role allocation and reasoning for that. But we’ll come back to that. But one of the other things around attention, and essentially, the transformer models that underlie generative AI are they’re founded on how it is they allocate attention, the self-attention models. But just looking at a human perspective, you’ve, also had some very interesting findings around the role of gender in collective intelligence and human groups. High level just interested in how gender roles play out, in collective attention.
Anita: Yeah, no, it’s been an ongoing learning. So the relationship between gender composition and collective intelligence was not initially something we were focused on. So in our early studies, we observed a correlation between the proportion of women in a group and collective intelligence. And at first, we thought, oh, maybe that’s spurious, or, maybe there’s something about our sample or something. But it has continued to show up. And even in the meta-analysis that we published a couple of years ago, in PNAS that had over a thousand groups in our data set the correlation was still there.
A lot of in a lot of the studies, though, it’s at least partially explained and, and some cases fully mediated by other qualities of the members, specifically social perceptiveness, or the ability to pick up on subtle cues and draw inferences about what others are thinking or feeling or to anticipate how they might respond to something, and so on and use that information to help facilitate the work of the group. And so we’ve, in more recent years, done a series of studies to try to further unpack both how collective cognition forms, but also maybe the roles that attention might play. And so we do see that when we have groups with people who are more socially perceptive, not only do the conversational patterns tend to be more productive, and more, I guess, supportive of collective intelligence. Also we see a variety of other ways that it manifests in behavior, such as various forms of behavioral synchrony, facial expression, synchrony, vocal cue synchrony, but also synchronizing activity patterns together.
So in some of our studies, we’ve observed this quality we call burstiness. Communication patterns where both teams that are together face to face as well as teams that are distributed even across the world, in some cases, when the teams that are more collectively intelligent, are better at sort of picking up on and being responsive to each other and concentrating their exchanges in shorter bursts. So you could have two teams that have communicated the same amount, but one team is much more bursty and has more concentrated exchanges of information. And another team in the other team isn’t. The more bursty team is almost always more collectively intelligent. And so we found that to, you know, also correlate with gender composition of teams with social perceptiveness in teams. And to be something that is facilitated by actually having a leader having a stable hierarchy in the team seems to facilitate this process because all the individuals in the team will orient to the leader for the cues on okay, what are we doing now? Or are we talking now? Or are we working individually, etc. Whereas, in teams that don’t have a stable leader, or especially in teams, where the individuals are competitive with each other to be in charge, you don’t have that synchrony. There’s much more competitive behavior, and a lot of interruption in the speaking patterns, and generally less collective intelligence.
Ross: Right. Well, this actually leads us, I think, to the roles of AI, and collective intelligence in facilitating human interaction. Now, obviously, there are many roles for AI in collective intelligence. But the first step is to say, well, I’ve got a bunch of humans who are demonstrating some degree of collective intelligence. So how can AI be used to facilitate human interaction in a way that supports collective intelligence?
Anita: I think there are a variety of things we’ve looked at already, some that have worked, some that haven’t. And then some that are studies underway. So, one thing we learned in our early studies is that it was easier for technology to mess up collective intelligence than to enhance it, in the sense that humans don’t really want machines to tell them how to interact with each other. It’s easier to overstep in that situation, and so we have a paper that came out, I believe, a little earlier this year, in MIS quarterly, looking at nudges. And so having technology-based nudges picking up on cues about how an interaction is going or how a team is performing, and then just nudging certain things.
And an important thing about a nudge is that the human maintains their autonomy. That’s a definition to a nudge, it’s sort of increasing the probability of a particular decision, but not preventing any alternatives. And so, we have been developing behavioral indicators of collective intelligence in a team over time. And then on that basis, different suggestions could be formed. So for example, if a team is working together on a problem, and these members may not know each other very well. And it’s clear to the system and the underlying pattern of things that there are people who know things that aren’t, their knowledge isn’t being used, or the wrong people are doing, you know, different parts of the task. And so a nudge in this is the main one that was successful in our study was kind of saying, ‘Well, why don’t you pause a moment, and just step back and think about who’s doing what and how you have things allocated, and whether you want to consider any changes.’
And so essentially, sort of nudging the team members, the human members to talk to each other, was by far the most successful attempt, anything we did that was heavier-handed, in some cases, backfired. People sort of withdrew or had different reactions to what the facilitators were doing. So one principle we kind of took from that was, that the things that AI would probably be the most useful for are things that might either facilitate or reinforce human collaboration with each other, not try to a term that we started using on one project, DARPA was joystick, not joystick, the whole thing where it’s like, okay, now Ross, you say this, and then Anita, you say that, or whatever, the different levers might be, but rather to maybe start by reinforcing and getting the group to develop better collaboration together. And so that I think would be a really low-hanging fruit in a really good way to try to think about deploying AI.
With generative AI, and specifically large language models. I mean, there are many exciting possibilities. And among them is the way that it could become something that’s maybe a team, more of a teammate than a lot of human-computer systems human team interaction studies tend to see. And so if you think about, for example, a lot of us will ask Google things that we would never ask our teammate, right? If we expanded that notion and instead had one of these generative AI teammates, where not only maybe that I could ask that teammate something and feel less intimidated about being judged. What this teammate’s opinion is of me. But you could also imagine how this teammate could facilitate the interaction of the human team members by, you know, sort of passing on information or prompting a conversation that might not have happened, you could even imagine if there was a conflict between two teammates, and they’re talking to their AI teammate, if the AI teammate couldn’t help facilitate some sort of a resolution, help each of them take a different perspective, for example, or do things to try to help heal this situation. So I think those are exciting opportunities as well.
Ross: Fantastic. So I think in a way two levels that you’ve laid out there, one is where clearly generative AI does an analysis of all of the communication patterns in order to be able to surface these nudges. And then, so all the communication patterns all going on, and then as a result of that, being able to suggest these various suggestions that might come up with so you’ve begun to identify some nudges that are successful. So presumably, over time with more data, we could refine or improve the ways in which, generative AI monitoring, as it were communication patterns could get better or better at being able to nudge thos things that would improve collective intelligence.
Anita: Yeah, absolutely. And I mean, I realize people I mean, there’s, of course, flags about privacy all over this right, which is not a trivial concern. I’ve been surprised by how much.
So most of the analyses I was mentioning where we were doing this, we had, we didn’t even touch the content of what people were saying, right? It was really based on patterns, who’s contributing, who’s not contributing, who’s responding, who are we not hearing from at all?
Ross: Or the wage level?
Anita: Exactly. And so even that I realize is sensitive. But I think it’s important to, you know, think about how, rather than taking all the information we possibly can just because we can, figuring out what we really need to be able to do something helpful? And how much does it really improve what we can do by invading people’s privacy further? So I think that’s really important, and it’s in a very important question, obviously, that comes into all of this.
Ross: Yeah, well, I think that’s, I think it’s a great point where, in a way, even if you just separate out those layers of just looking at the metadata, I think communication patterns, and then communication content, and a few, and probably the vast majority of the value is at the pattern level as opposed to the content level.
Anita: That’s right. That’s right. And or if you can capture sentiment, which you can, you know, and just, you know, that’s the only information that’s used, not anything about the content.
Ross: So, you started to talk about the next thing, which is an AI teammate. I loved it. So I suppose that’s another frame. And then the next phase beyond that is where you have what you describe as a multi-agent system with both human and AI, more participants, let’s call them. And so from looking at, I suppose, looking at the human communication patterns and finding nudges to be able to bring in an AI participant, or single AI participant, which as you say, can play a facilitative role or can play other roles in information brokering or other things which can be designed to enhance a team capabilities. So then what happens? Where are we in terms of looking at those next steps where we look at multi human, multi AI, participants in a collectively intelligent system?
Anita:I think, certainly these things are being modeled or simulated. I think that that, to me feels like just kind of a, I don’t know, an explosion, I guess. But I was gonna say, an expansion on what I was talking about with the one agent, maybe as part of a three-person team just in our little hypothetical example. But you could easily imagine that there could be different agents playing different roles. Even now, there are, you know, settings where some studies and even exercises in the classroom that we do have agents that are just content-based, and there are others that are watching the process and intervening in the process of what’s happening.
You could have, especially when we start talking about issues of trust, it could be that each person in the team has their own agent, almost like their Jimny Cricket, if you will, who is there, you know, sort of like assisting them and maybe talking with the other agents to figure out how we’re going to help this poor team? Yeah, I mean, it kind of expands considerably. And I think the other opportunity there is, a lot of times we pull together diverse teams in order to get a variety of perspectives. But there’s also an exciting opportunity potentially, to be able to get those perspectives through agents, right? If we know the right kind of characteristics or backgrounds that we need to simulate. And we know that we can faithfully do that, you know, that also provides sort of a mechanism for pulling in those perspectives and integrating them so that perhaps the solutions we come up with would be better. Now, that doesn’t mean it gets rid of the need for diversity in the team, because I think the human teammates are going to have different ways that they’re going to draw things out of the artificial intelligence, right? And so you still benefit from having diversity within the team, but then you have access to even greater diversity. So I think that is also exciting when we talk about solving very complex problems, especially all the multifaceted problems that are the toughest, like climate change, and so on.
Ross: Absolutely. That’s, that’s one of the ways in which I use generative AI is saying, ‘Alright, well, we’ve got these perspectives so far, what perspectives are missing?’ And they can find some perspectives, which haven’t been brought to bear yet.
Anita: Yeah, absolutely. And I think leveraging that and identifying, Okay, how about if I had this set of values? Or if I was kind of delineating what the things are for this particular problem that might really change how people perceive a particular solution.
Ross: So one of the phrases uses this idea of integrated collaboration between humans and AI. So what does that speak to? How do we truly integrate that collaboration between humans and AI?
Anita: I think it’s happening little by little even now, I mean, in very small ways, you know, when different assistant things pop up, and remind me or whatever helps us do something that we couldn’t do otherwise. We are working on a project right now through the NSF AI institute called AI caring, which is focused on the problem of helping the elderly age in place.
The portion of it that my team is working on is focusing on how we set up robust teaming in the caregiver networks that are involved, who often don’t even know that they’re part of a team. They’re all the people that help this, say, this elderly person with mild cognitive impairment. It’s a neighbor, it’s a nurse, it’s a, you know, family member, etc. But there’s always, if any, if anybody had to help a family member or somebody who’s in the stage of their life, there are all kinds of pieces of information that different people will know, and they should be passed on. But there’s no really good way to do it.
And so what we’re working on is a tool that could, maybe even be on somebody’s cell phone, where, ‘Okay, I’m a neighbor, and I’m taking, you know, Mr. Dawson out to lunch today, just to give his wife a break.’ And then, you know, this, this agent would maybe pass on some things to me that I should know about, you know, he’s been not steady on his feet, he might fall over, or he needs to make sure he takes this medicine before he eats, etc. And then maybe during my interaction, maybe there are some things I’m concerned about, and I can let our helper agent know. And then this agent can pass that along and can help coordinate or maybe even flag something in a pattern that is coming up that different people are noting, but nobody is connecting. And so I think bringing the tools that we have into our networks to help humans coordinate and pass along information is going to be one of the key ways that we can benefit, I think from really integrating the human and the AI, you know, capability. Essentially.
Ross: That’s a lovely application qne illustration of it. I think very, very grounded and very, very human. So, I like to pull back to the very big picture, rather than collective intelligence. I remember stuff happening in the 90s. And they’re in collective intelligence, there’s a lot of, I suppose, looking at the architectures of collective intelligence, which have progressed quite a lot over the decades. We know of course, as AI is something which we can, play a role, be a, you know, human peer equivalent as we look at peer roles, as well as some of how we can bring these together. And, of course, you know, a lot of research from you and your colleagues, obviously, the MIT Center for Collective Intelligence, many researchers all over the world. So, I’d like to just sort of pull back to reflect on where we’ve got to and where we are. What are the frontiers in coming years and collective intelligence?
Anita: Well, I just see the opportunities for a whole variety of ways that the new capabilities of artificial intelligence can amplify and help amplify the abilities of the humans and so one way would be we talked a little bit about being an intermediary in a conflict but even in a conversation. So, I don’t know what it’s like in your part of the world now, but for us, everybody’s so busy, and there are so many different things happening at the same time, it’s even hard to have a meeting. And we’ve tried to have meetings too often because we don’t know any better way to collaborate. And we could imagine, actually, maybe there’s a time coming when we don’t need to have a meeting.
We have, part of our teammates, these agents who go talk with each person and get their perspective, and, and portray it for others and get their perspective and integrate it and kind of asynchronously help us have this discussion, right, where, and probably do it better than we do for each other — they could be great listeners, they could ask great questions, they could, you know, do all kinds of things that we know, humans can be not great at, especially if there are other things in the situation where they’re anxious, or, they’re in conflict, or whatever the case might be. I mean, I think that, and probably, you know, other aspects of technology will develop too. And so we won’t be sitting in front of a screen and looking at a camera, we’ll be, I’ll just be sitting in my chair, and you’ll be sitting in the chair across for me and we’ll be having these interactions without having to synchronize for them entirely. So, that’s kind of where I see things going, I hope that they go in a way that, again, kind of strengthens and reinforces, you know, human capability and human connection, versus completely replacing it. But, there’s always a danger of that, of course.
Ross: Yes, but I think the intent is what is going to bring us to the, you know, truly human-centered approach where AI compliments us, as opposed to replacing us and that’s all it’s all how we choose to go about it.
Anita: Yeah. Yes, totally agree. So I hope everybody’s intentions can be in the right place.
Ross: Thank you so much for your time your insight and all of your work I need. I think it’s extremely important and has taken us to better places.
Anita: Well, I hope so too. Thanks. Thanks for your questions. I really enjoyed our conversation.
Podcast: Play in new window | Download